Challenge: Putting It All Together
In this challenge, you will apply everything you learned throughout the course from data preprocessing to training and evaluating the model.
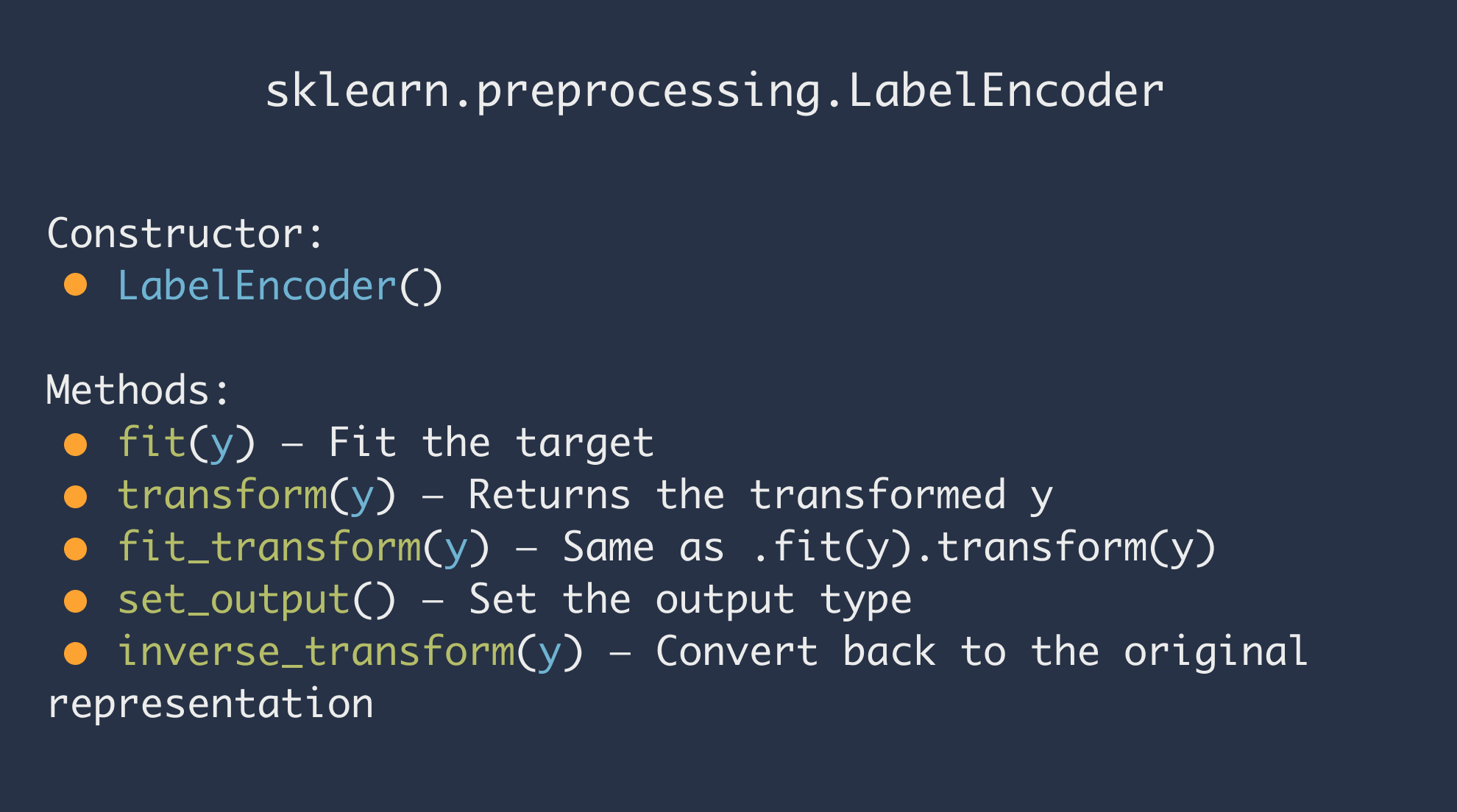
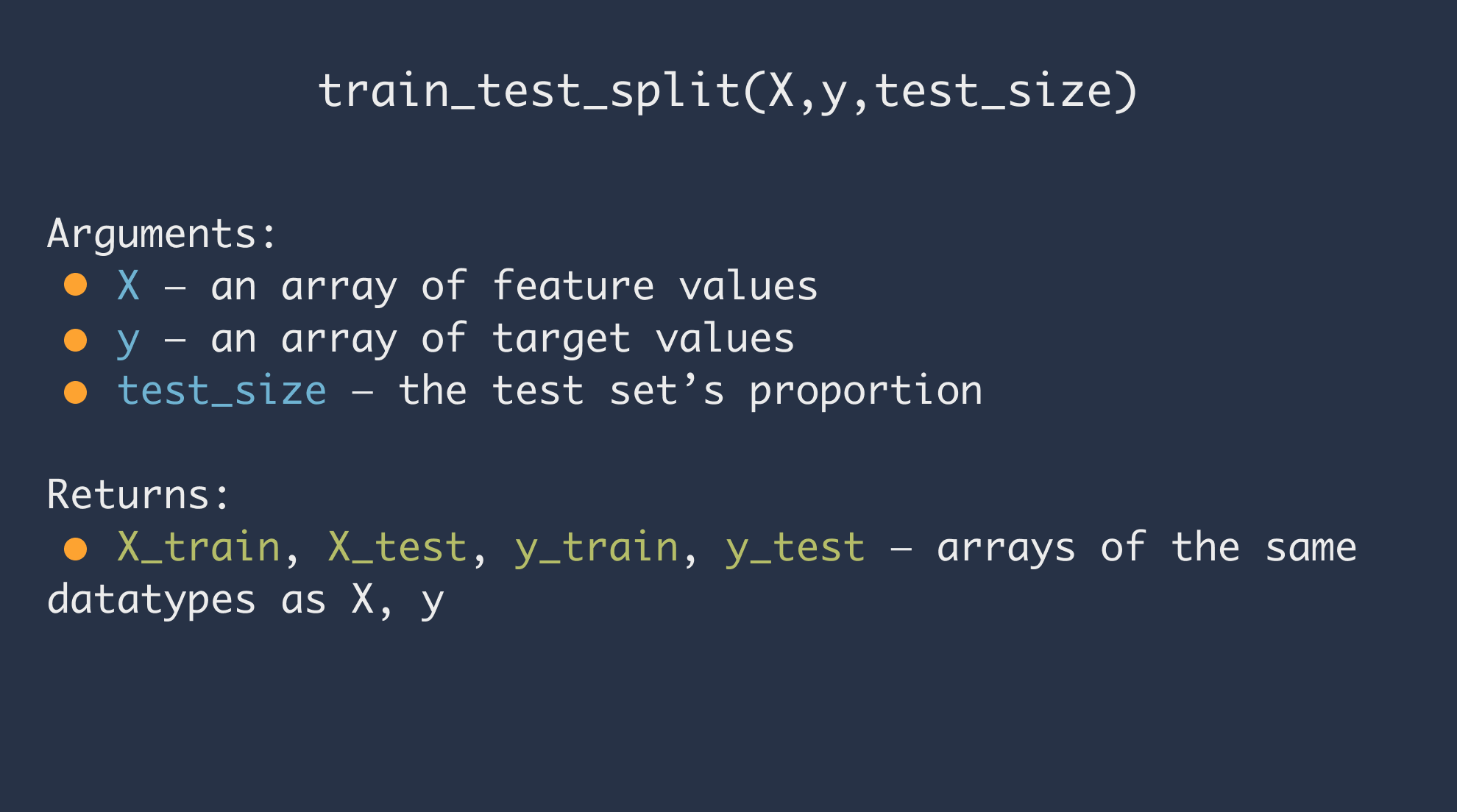
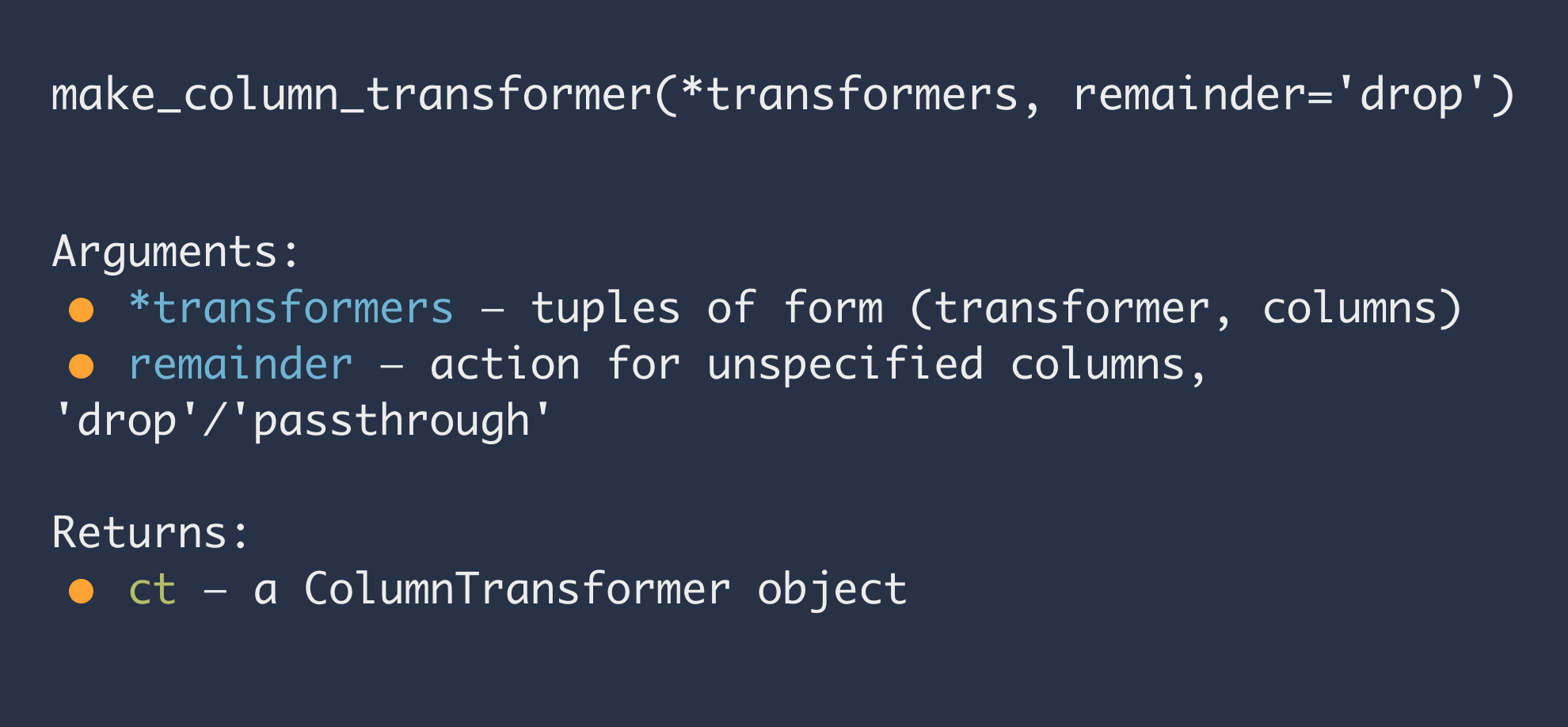
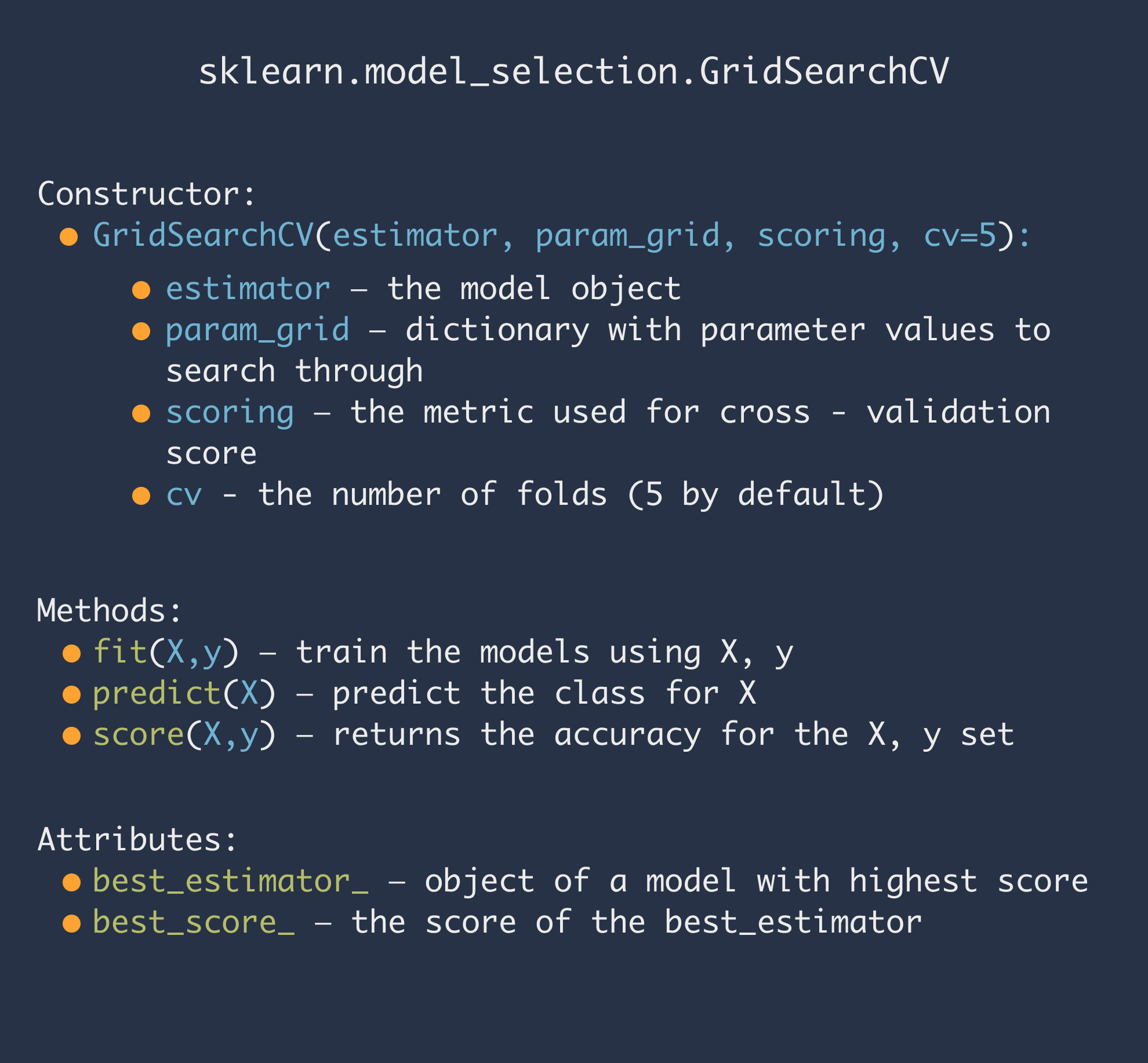
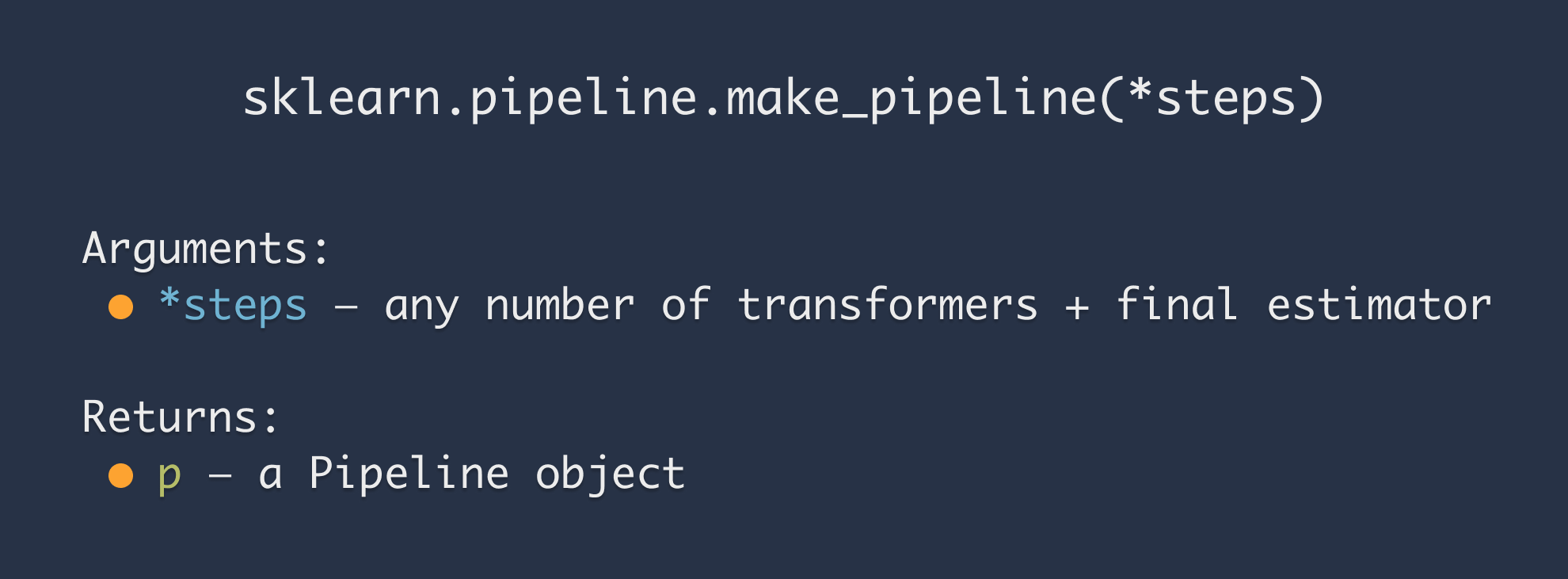
Compito
Swipe to start coding
- Encode the target.
- Split the data so that 33% is used for the test set and the remainder for the training set.
- Make a
ColumnTransformer
to encode only the'island'
and'sex'
columns. Make sure the others columns remain untouched. Use a proper encoder for nominal data. - Fill the gaps in a
param_grid
to try the following values for the number of neighbors:[1, 3, 5, 7, 9, 12, 15, 20, 25]
. - Create a
GridSearchCV
object with theKNeighborsClassifier
as a model. - Construct a pipeline that begins with
ct
as the first step, followed by imputation using the most frequent value, standardization, and concludes withGridSearchCV
as the final estimator. - Train the model using a pipeline on the training set.
- Evaluate the model on the test set. (Print its score)
- Get a predicted target for
X_test
. - Print the best estimator found by
grid_search
.
Soluzione
99
1
2
3
4
5
6
7
8
9
10
11
12
13
14
15
16
17
18
19
20
21
22
23
24
25
26
27
28
29
30
31
32
33
34
35
36
import pandas as pd
from sklearn.impute import SimpleImputer
from sklearn.preprocessing import OneHotEncoder, LabelEncoder, StandardScaler
from sklearn.compose import make_column_transformer
from sklearn.pipeline import make_pipeline
from sklearn.neighbors import KNeighborsClassifier
from sklearn.model_selection import GridSearchCV, train_test_split
df = pd.read_csv('https://codefinity-content-media.s3.eu-west-1.amazonaws.com/a65bbc96-309e-4df9-a790-a1eb8c815a1c/penguins.csv')
# Removing rows with more than 1 null
df = df[df.isna().sum(axis=1) < 2]
# Assigining X, y variables
X, y = df.drop('species', axis=1), df['species']
# Encode the target
label_enc = LabelEncoder()
y = label_enc.fit_transform(y)
# Make a train-test split
X_train, X_test, y_train, y_test = train_test_split(X, y, test_size=0.33)
# Create the ColumnTransformer for encoding features
ct = make_column_transformer((OneHotEncoder(), ['island', 'sex']), remainder='passthrough')
# Make a param_grid for the grid search and initialize the GridSearchCV object
param_grid = {'n_neighbors': [1, 3, 5, 7, 9, 12, 15, 20, 25],
'weights': ['distance', 'uniform'],
'p': [1, 2]
}
grid_search = GridSearchCV(KNeighborsClassifier(), param_grid)
# Make a Pipeline of ct, SimpleImputer, StandardScaler, and GridSearchCV
pipe = make_pipeline(ct, SimpleImputer(strategy='most_frequent'), StandardScaler(), grid_search)
# Train the model
pipe.fit(X_train, y_train)
# Print score
print(pipe.score(X_test, y_test))
# Print predictions
y_pred = pipe.predict(X_test) # Get encoded predictions
print(label_enc.inverse_transform(y_pred[:5])) # Decode predictions and print 5 first
# Print the best estimator
Tutto è chiaro?
Grazie per i tuoi commenti!
Sezione 4. Capitolo 10
99
1
2
3
4
5
6
7
8
9
10
11
12
13
14
15
16
17
18
19
20
21
22
23
24
25
26
27
28
29
30
31
32
33
34
35
36
37
import pandas as pd
from sklearn.impute import SimpleImputer
from sklearn.preprocessing import OneHotEncoder, LabelEncoder, StandardScaler
from sklearn.compose import make_column_transformer
from sklearn.pipeline import make_pipeline
from sklearn.neighbors import KNeighborsClassifier
from sklearn.model_selection import GridSearchCV, train_test_split
df = pd.read_csv('https://codefinity-content-media.s3.eu-west-1.amazonaws.com/a65bbc96-309e-4df9-a790-a1eb8c815a1c/penguins.csv')
# Removing rows with more than 1 null
df = df[df.isna().sum(axis=1) < 2]
# Assigining X, y variables
X, y = df.drop('species', axis=1), df['species']
# Encode the target
label_enc = LabelEncoder()
y = ___
# Make a train-test split
X_train, X_test, y_train, y_test = ___(X, y, ___=0.33)
# Create the ColumnTransformer for encoding features
ct = ___((___(), ___, ___=___)
# Make a param_grid for the grid search and initialize the GridSearchCV object
param_grid = {'n_neighbors': ___,
'weights': ['distance', 'uniform'],
'p': [1, 2]
}
grid_search = ___
# Make a Pipeline of ct, SimpleImputer, and StandardScaler
pipe = ___
# Train the model
___
# Print score
print(pipe.___)
# Print predictions
y_pred = pipe.___ # Get encoded predictions
print(label_enc.inverse_transform(y_pred[:5])) # Decode predictions and print 5 first
# Print the best estimator
print(grid_search.___)
Chieda ad AI
Chieda pure quello che desidera o provi una delle domande suggerite per iniziare la nostra conversazione