February vs July Average Temperatures
Well, as you remember, there are no 100% correct answers to clustering problems. For the last task you solved it seems like 5 clusters might be a good option.
Let's visualize the results of clustering into 5 groups by building the scatter plot for average February vs July temperatures, which are one of the coldest and hottest months respectively.
Taak
Swipe to start coding
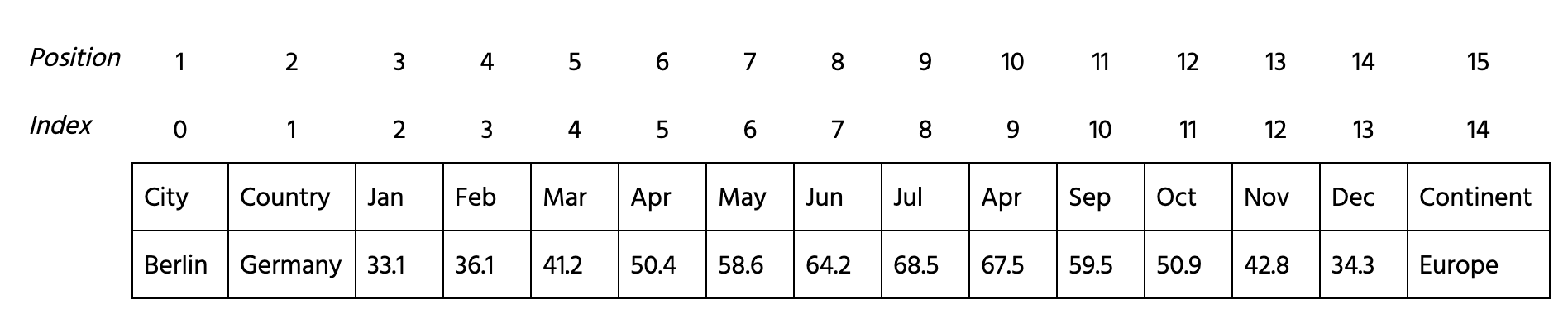
- Create a
KMeans
model namedmodel
with 5 clusters. - Fit the numerical columns of
data
(2 - 13 indices) tomodel
. - Add the
'prediction'
column to thedata
DataFrame with predicted bymodel
labels. - Build a scatter plot of average
'Feb'
vs'Jul'
temperatures, having each point colored with respect to the'prediction'
column of thedata
DataFrame.
Oplossing
Was alles duidelijk?
Bedankt voor je feedback!