Basic Linear Algebra with NumPy
Linear algebra is a fundamental branch of mathematics that plays a crucial role in various fields, including machine learning, deep learning, and data analysis.
Vectors and Matrices
In linear algebra, a vector is an ordered set of values. 1D NumPy arrays can efficiently represent vectors. A matrix is a two-dimensional array of numbers, which can be represented by a 2D array in NumPy.
We have already covered vector and matrix addition and subtraction, as well as scalar multiplication, in the "Basic Mathematical Operations" chapter. Here, we will focus on other operations.
Transposition
Transposition is an operation that flips a matrix over its diagonal. In other words, it converts the rows of the matrix into columns and the columns into rows.
You can transpose a matrix using the .T
attribute of a NumPy array:
Dot Product
The dot product is perhaps the most commonly used linear algebra operation in machine and deep learning. The dot product of two vectors (which must have an equal number of elements) is the sum of their element-wise products. The result is a scalar:
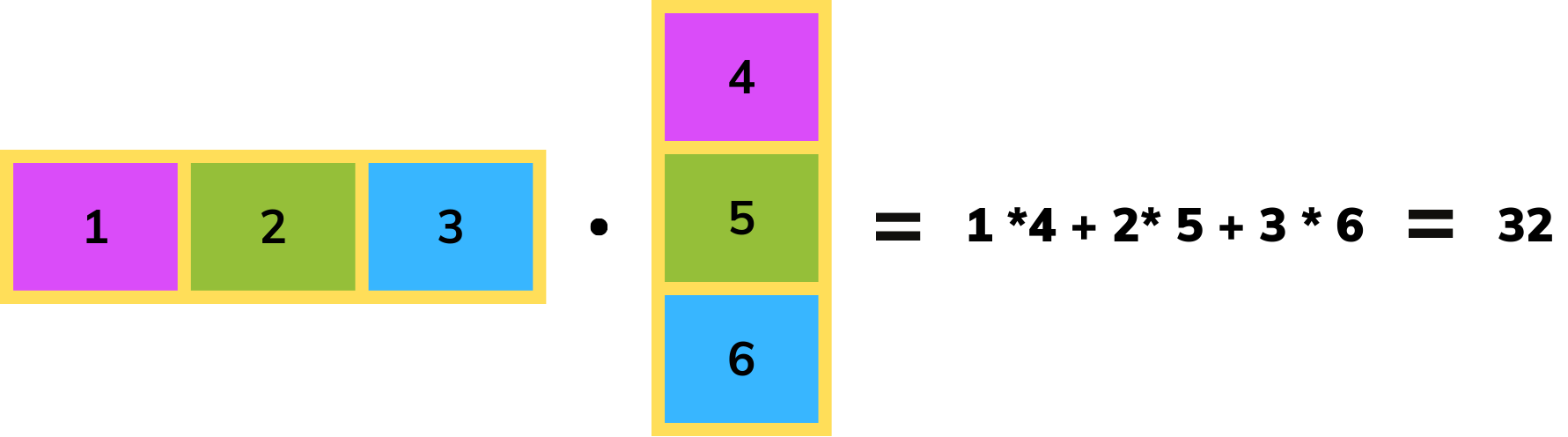
Matrix Multiplication
Matrix multiplication is defined only if the number of columns in the first matrix is equal to the number of rows in the second matrix. The resulting matrix will have the same number of rows as the first matrix and the same number of columns as the second matrix.
Here is a visualization of matrix multiplication:
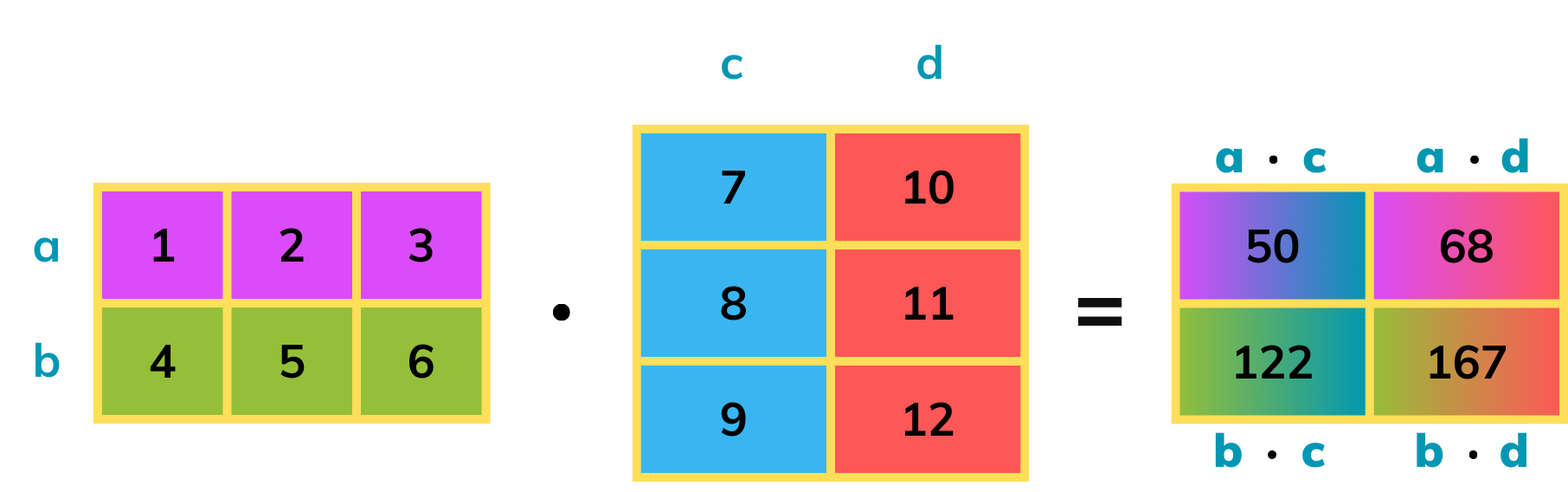
As you can see, every element of the resulting matrix is the dot product of two vectors. The row number of the element corresponds to the number of the row vector in the first matrix, and the column number corresponds to the number of the column vector in the second matrix.
The number of columns in the first matrix must be equal to the number of rows in the second matrix, as the dot product requires the two vectors to have the same number of elements.
Dot Product and Matrix Multiplication in NumPy
NumPy provides the dot()
function for both the dot product and matrix multiplication. This function takes two arrays as its arguments.
However, you can also use the @
operator between two arrays to achieve the same results.
Let’s look at an example:
Note
When the right argument in matrix multiplication in NumPy is a vector (1D array), it is promoted to a matrix by appending a
1
to its dimension. When the left argument in matrix multiplication is a vector, it is promoted to a matrix by prepending a1
to its dimensions.
For example, a vector with 4
elements will be treated as a 4x1
matrix if it is the right argument or a 1x4
matrix if it is the left argument.
Tarefa
exam_scores
contains simulated exam scores of three students (each row corresponds to a student) from three subjects (each column corresponds to a subject).
Each subject exam should be multiplied by the respective coefficient, and the resulting scores for each student should be added to get the final score for each student.
Your task is to calculate the dot product between exam_scores
and coefficients
using the appropriate operator.
Tudo estava claro?
Conteúdo do Curso
Ultimate NumPy
3. Commonly used NumPy Functions
Ultimate NumPy
Basic Linear Algebra with NumPy
Linear algebra is a fundamental branch of mathematics that plays a crucial role in various fields, including machine learning, deep learning, and data analysis.
Vectors and Matrices
In linear algebra, a vector is an ordered set of values. 1D NumPy arrays can efficiently represent vectors. A matrix is a two-dimensional array of numbers, which can be represented by a 2D array in NumPy.
We have already covered vector and matrix addition and subtraction, as well as scalar multiplication, in the "Basic Mathematical Operations" chapter. Here, we will focus on other operations.
Transposition
Transposition is an operation that flips a matrix over its diagonal. In other words, it converts the rows of the matrix into columns and the columns into rows.
You can transpose a matrix using the .T
attribute of a NumPy array:
Dot Product
The dot product is perhaps the most commonly used linear algebra operation in machine and deep learning. The dot product of two vectors (which must have an equal number of elements) is the sum of their element-wise products. The result is a scalar:
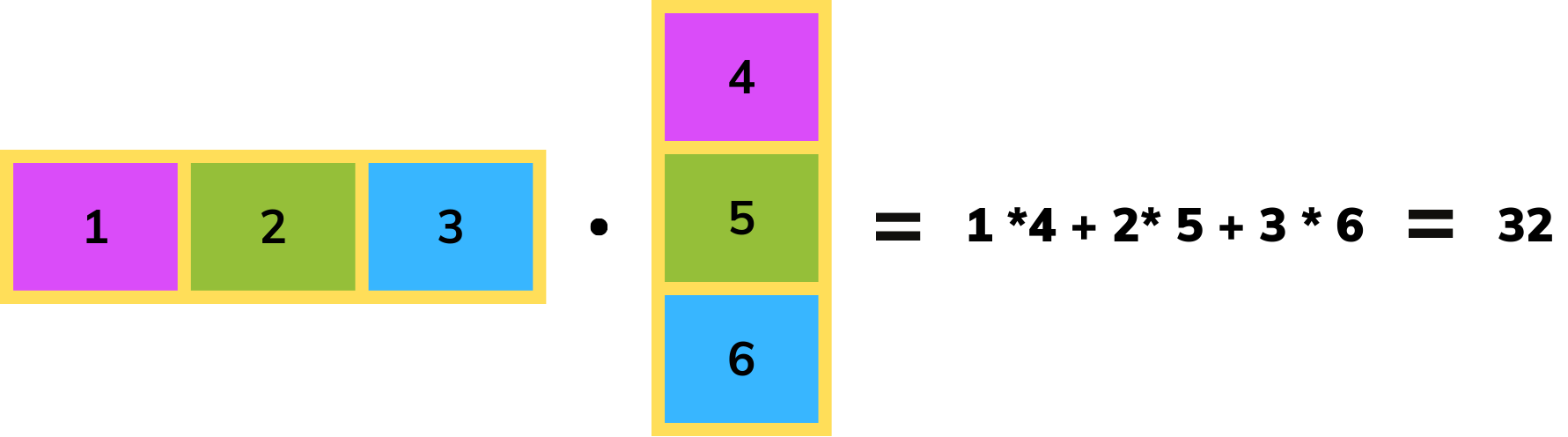
Matrix Multiplication
Matrix multiplication is defined only if the number of columns in the first matrix is equal to the number of rows in the second matrix. The resulting matrix will have the same number of rows as the first matrix and the same number of columns as the second matrix.
Here is a visualization of matrix multiplication:
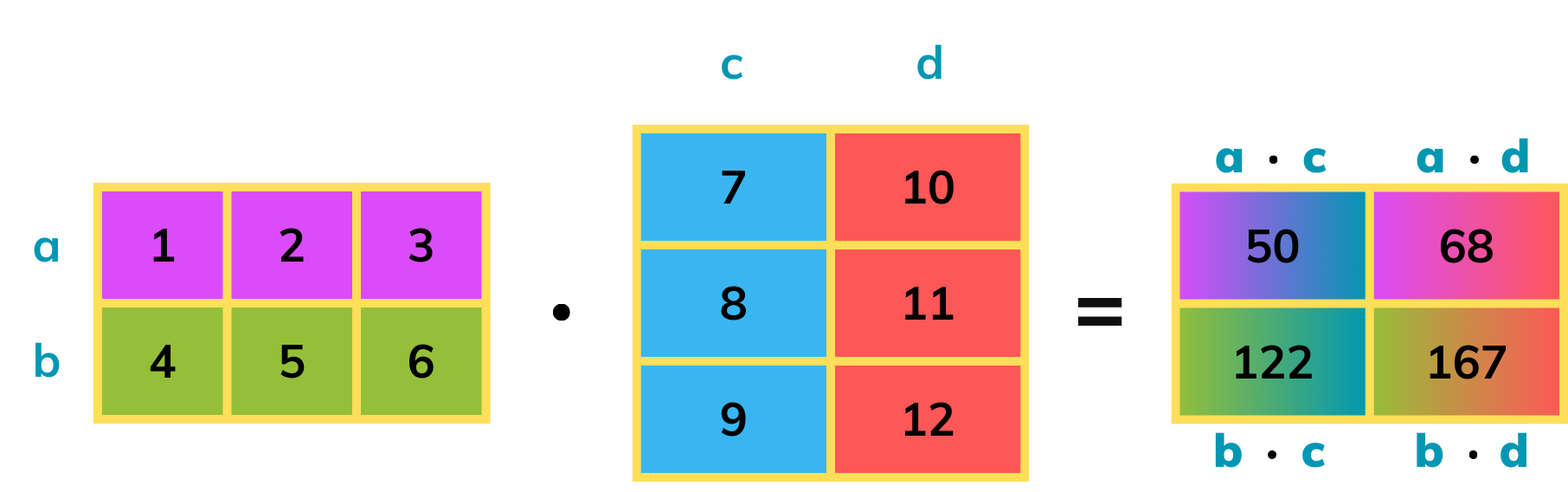
As you can see, every element of the resulting matrix is the dot product of two vectors. The row number of the element corresponds to the number of the row vector in the first matrix, and the column number corresponds to the number of the column vector in the second matrix.
The number of columns in the first matrix must be equal to the number of rows in the second matrix, as the dot product requires the two vectors to have the same number of elements.
Dot Product and Matrix Multiplication in NumPy
NumPy provides the dot()
function for both the dot product and matrix multiplication. This function takes two arrays as its arguments.
However, you can also use the @
operator between two arrays to achieve the same results.
Let’s look at an example:
Note
When the right argument in matrix multiplication in NumPy is a vector (1D array), it is promoted to a matrix by appending a
1
to its dimension. When the left argument in matrix multiplication is a vector, it is promoted to a matrix by prepending a1
to its dimensions.
For example, a vector with 4
elements will be treated as a 4x1
matrix if it is the right argument or a 1x4
matrix if it is the left argument.
Tarefa
exam_scores
contains simulated exam scores of three students (each row corresponds to a student) from three subjects (each column corresponds to a subject).
Each subject exam should be multiplied by the respective coefficient, and the resulting scores for each student should be added to get the final score for each student.
Your task is to calculate the dot product between exam_scores
and coefficients
using the appropriate operator.
Tudo estava claro?