Cursos relacionados
Ver Todos os CursosIntermediário
ML Introduction with scikit-learn
Machine Learning is now used everywhere. Want to learn it yourself? This course is an introduction to the world of Machine learning for you to learn basic concepts, work with Scikit-learn – the most popular library for ML and build your first Machine Learning project. This course is intended for students with a basic knowledge of Python, Pandas, and Numpy.
Avançado
Introduction to Neural Networks
Neural networks are powerful algorithms inspired by the structure of the human brain that are used to solve complex machine learning problems. You will build your own Neural Network from scratch to understand how it works. After this course, you will be able to create neural networks for solving classification and regression problems using the scikit-learn library.
Ethical Considerations in Deep Learning
Ethical Considerations
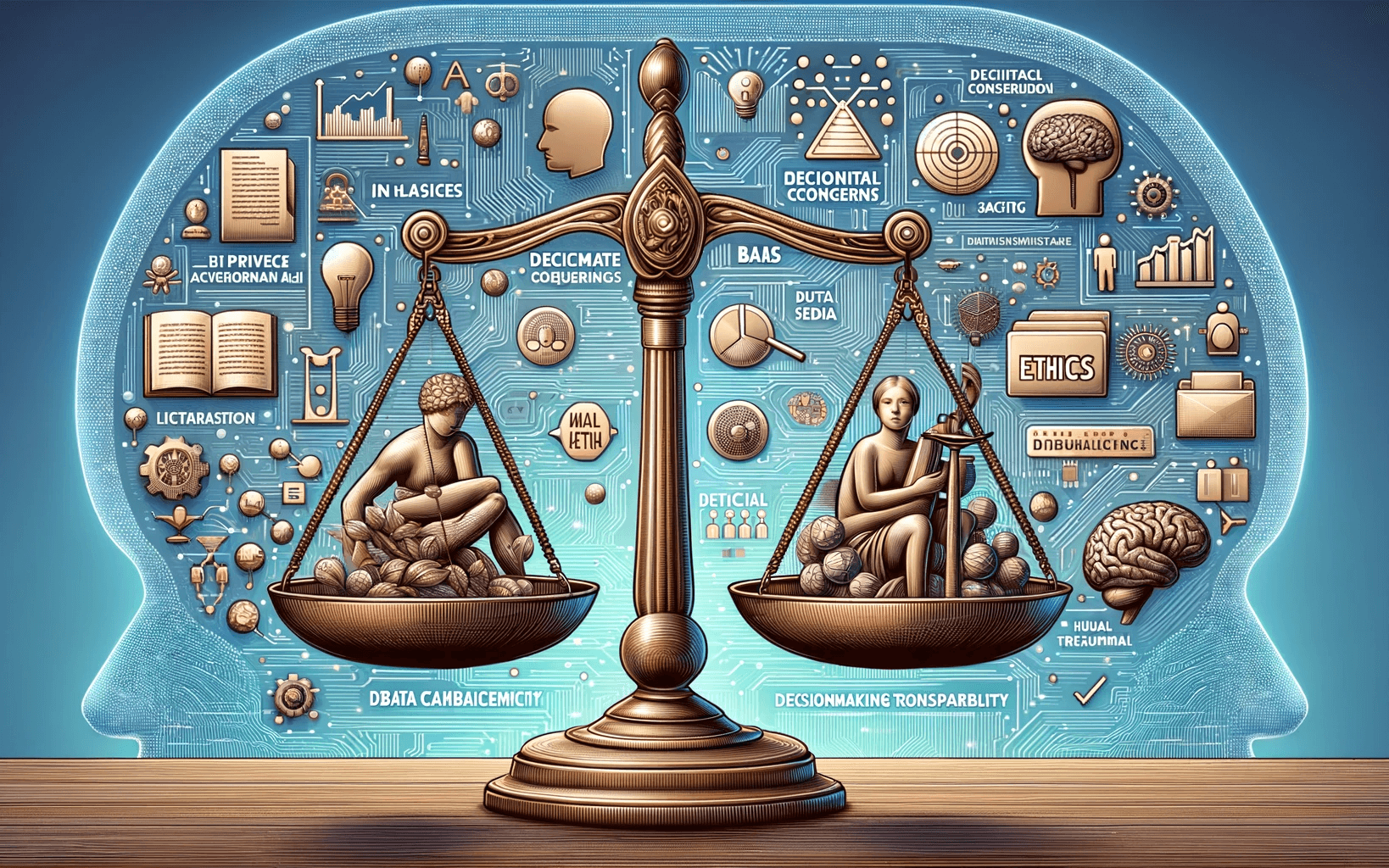
Introduction
Deep learning, a subset of artificial intelligence (AI), has made significant advancements in recent years, revolutionizing fields from healthcare to finance. However, these technological strides bring forth crucial ethical considerations. This article explores these ethical dimensions, highlighting the importance of responsibility in AI development.
Key Ethical Issues in Deep Learning
Bias and Fairness
- Core Concept: Bias in AI often arises from training data that reflects historical prejudices or lacks diversity.
- Examples:
- AI in recruitment software developing biases from historical hiring data, leading to discrimination.
- Loan approval algorithms trained on biased past data, resulting in unfair demographic preferences.
- Facial recognition systems failing to accurately identify individuals from certain racial backgrounds.
- AI in healthcare potentially overlooking diseases more prevalent in underrepresented groups.
- Mitigation: Utilize diverse datasets and incorporate fairness algorithms to reduce bias.
Data Privacy
- Core Concept: The ethical use of personal data in AI, particularly in sensitive sectors like healthcare, is a significant concern.
- Examples:
- Healthcare AI using patient data for disease prediction risking patient confidentiality breaches.
- Consumer data used in AI-driven marketing or financial models exposing individuals' private information.
- Genetic information used in research without patient consent.
- Mitigation: Implement strict data handling protocols, anonymize data, and ensure informed consent.
Transparency and Explainability
- Core Concept: AI systems often operate without transparency, leading to trust and accountability issues.
- Examples:
- Credit scoring AI not disclosing the basis for decision-making.
- AI in criminal justice used for risk assessment without explaining its conclusions.
- Automated content moderation systems on social media lacking transparency, leading to biased enforcement or censorship.
- Mitigation: Develop AI models with explainability in mind and enforce regulations requiring transparency.
Autonomy and Human Oversight
- Core Concept: Delegating critical decisions to AI systems without human oversight poses ethical risks.
- Examples:
- Autonomous military drones making target decisions.
- AI systems in healthcare making end-of-life care decisions independently.
- Mitigation: Ensure human-in-the-loop checkpoints and establish guidelines for human oversight in automated processes.
Accountability
- Core Concept: The diffusion of responsibility in AI systems presents challenges in establishing clear accountability.
- Examples:
- Self-driving cars involved in accidents raising questions about liability among manufacturers, algorithm designers, or owners.
- AI-driven financial advice leading to losses, with unclear responsibility between the algorithm, data, or institution.
- Erroneous medical diagnoses made by AI, leading to ambiguity in accountability.
- Mitigation: Develop clear legal frameworks and ethical guidelines to address accountability in AI systems.
Run Code from Your Browser - No Installation Required
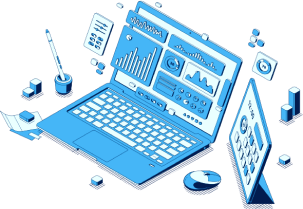
Addressing Ethical Challenges
Implementing Ethical Guidelines
The creation and enforcement of ethical guidelines are essential in guiding AI development. This involves setting standards that prioritize human dignity, privacy, and rights in the deployment of AI technologies.
For example, guidelines might dictate transparent data usage policies or mandate impact assessments to evaluate how AI systems affect different demographic groups. Such guidelines not only ensure compliance with ethical norms but also build public trust in AI technologies.
Diverse and Inclusive Data Sets
To combat inherent biases in AI, it's crucial to train models on data sets that are representative of diverse populations. This means including a wide range of ethnicities, genders, ages, and other sociodemographic factors. By doing so, AI models can more accurately reflect and serve the diverse needs of the global population.
For instance, in healthcare, diverse data sets can help in developing diagnostic tools that are effective across different racial and ethnic groups, thus ensuring equitable healthcare outcomes.
Continuous Monitoring and Auditing
Ongoing monitoring and auditing are key to maintaining ethical AI systems. This involves regularly reviewing AI algorithms and their outputs for biases, errors, or unethical behavior. Such practices can identify issues like discriminatory decision-making or privacy breaches. Monitoring should be coupled with mechanisms for accountability and rectification, ensuring that AI systems remain aligned with ethical standards throughout their lifecycle.
Collaboration Between Stakeholders
Addressing ethical concerns in AI requires a collaborative approach. This means bringing together AI developers, ethicists, legal experts, policymakers, and users to discuss and address the multifaceted ethical issues. Such collaboration can lead to the development of more balanced and fair AI systems.
For example, ethicists can provide insights on moral implications, while end-users can offer perspectives on how AI impacts their lives, leading to more responsible and inclusive AI solutions.
Education and Awareness
Educating stakeholders about the ethical aspects of AI is crucial. This includes training developers in ethical AI design, informing users about how AI decisions are made, and educating policymakers on the implications of AI technologies. Awareness programs can help demystify AI, dispel misconceptions, and promote informed discussions about the benefits and risks of AI. This education should extend to schools and universities, embedding ethical considerations in technology curricula to cultivate the next generation of responsible AI practitioners.
Conclusion
While deep learning offers immense potential, it is imperative to navigate its advancement with a strong ethical compass. Addressing these ethical considerations is crucial in ensuring that AI technologies benefit society as a whole, without compromising moral values or individual rights.
Start Learning Coding today and boost your Career Potential
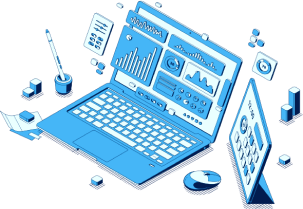
FAQs
Q: Can AI bias be completely eliminated?
A: While it's challenging to completely eliminate bias, efforts can be made to significantly reduce it through diverse data, ethical training, and regular audits.
Q: How can we ensure AI systems are transparent?
A: By designing AI systems with explainability in mind and implementing regulations that require transparency in AI decision-making.
Q: What role do governments play in AI ethics?
A: Governments can enact policies and regulations that set standards for ethical AI development and usage, protecting citizens from potential harms.
Q: Is it possible for AI to make ethical decisions?
A: AI's ability to make ethical decisions is limited by the data it is trained on and the algorithms' design. Human oversight is essential in scenarios requiring ethical judgment.
Q: How can the public stay informed about ethical AI?
A: Engaging with educational resources, staying updated with AI news, and participating in public discussions on AI ethics are ways the public can stay informed.
Cursos relacionados
Ver Todos os CursosIntermediário
ML Introduction with scikit-learn
Machine Learning is now used everywhere. Want to learn it yourself? This course is an introduction to the world of Machine learning for you to learn basic concepts, work with Scikit-learn – the most popular library for ML and build your first Machine Learning project. This course is intended for students with a basic knowledge of Python, Pandas, and Numpy.
Avançado
Introduction to Neural Networks
Neural networks are powerful algorithms inspired by the structure of the human brain that are used to solve complex machine learning problems. You will build your own Neural Network from scratch to understand how it works. After this course, you will be able to create neural networks for solving classification and regression problems using the scikit-learn library.
Revolution in Artificial Intelligence of 2024
Exploring the groundbreaking innovations that reshaped industries last year
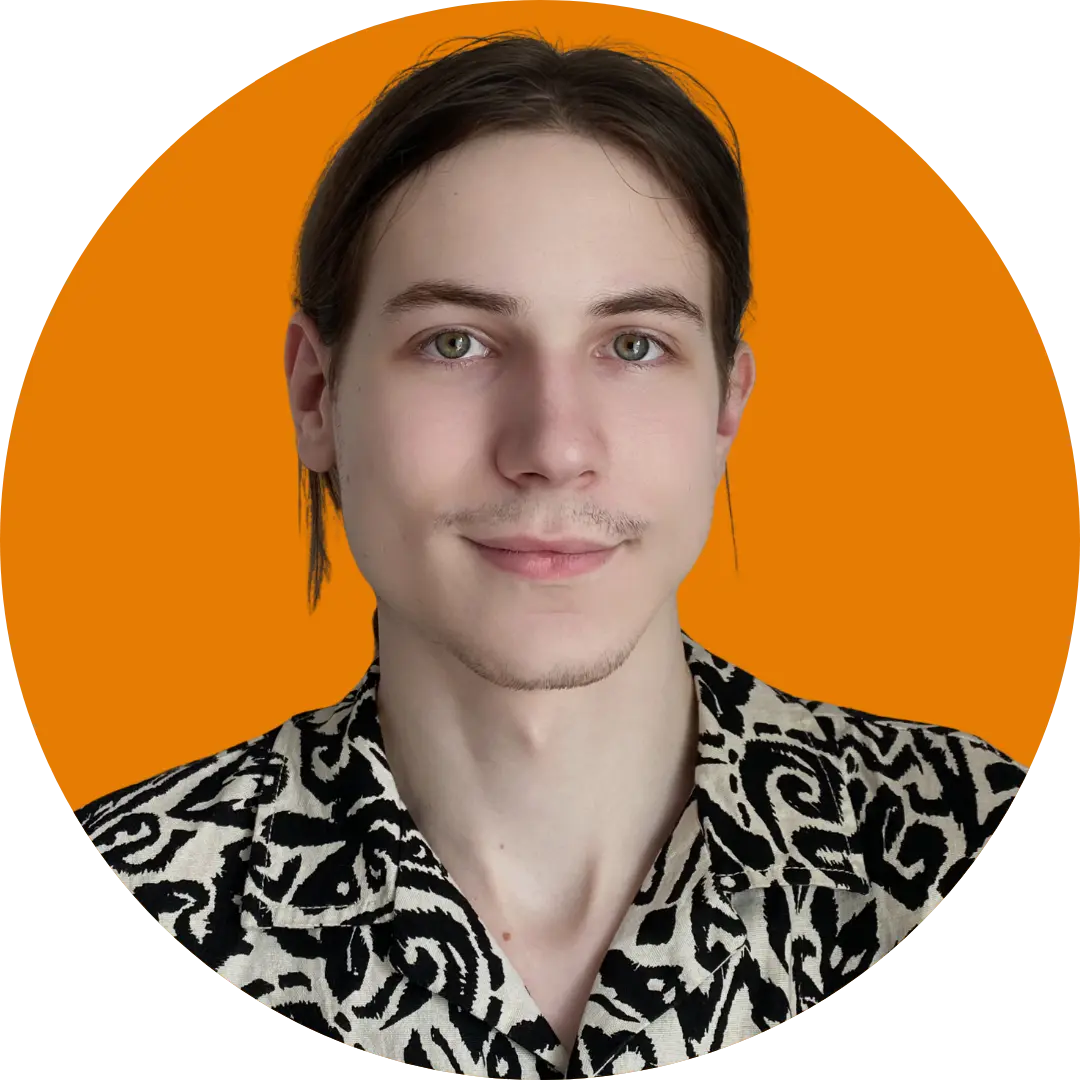
by Ihor Gudzyk
C++ Developer
Jan, 2025・8 min read
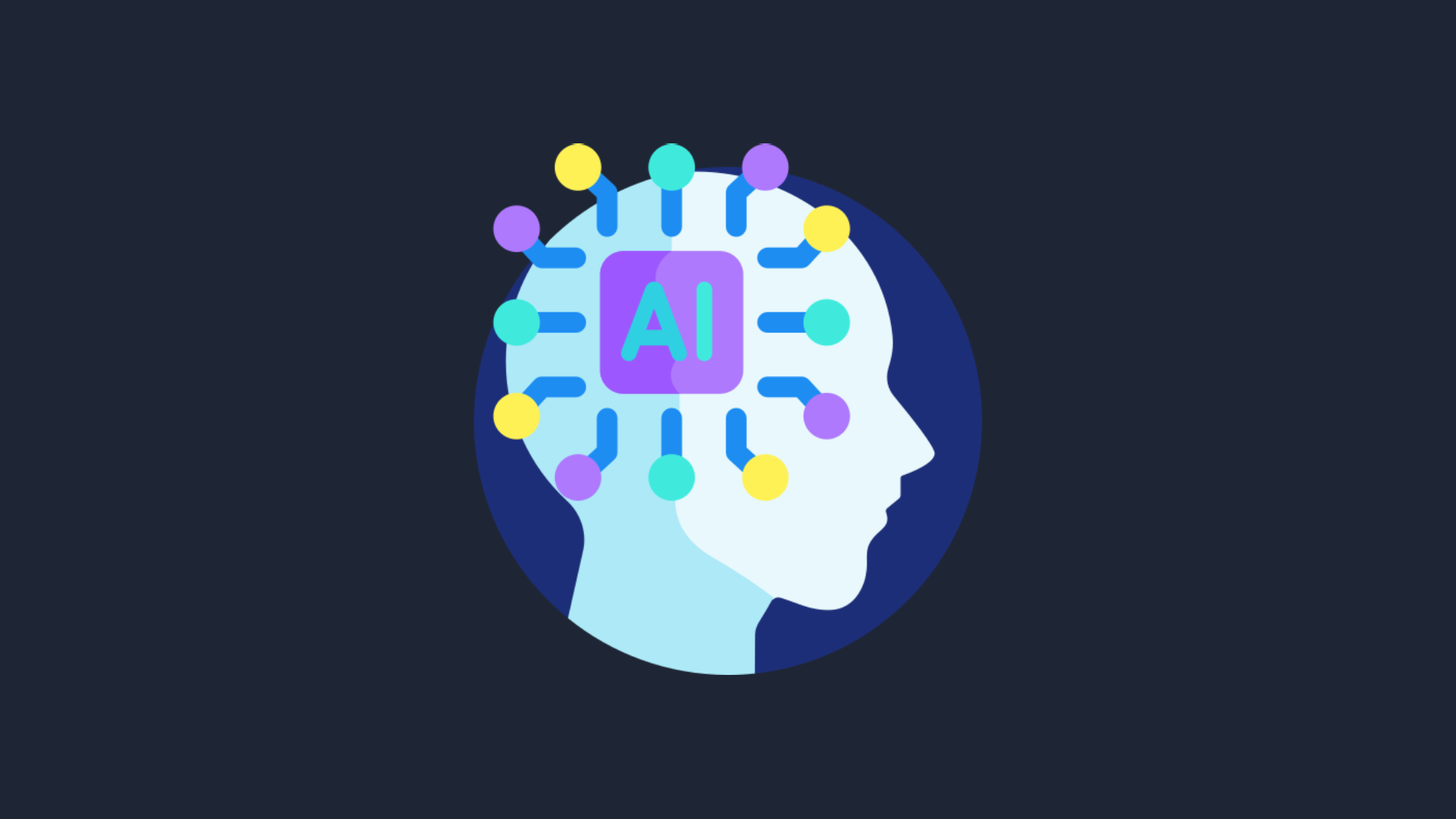
Fine Tuning vs Feature Extraction in Transfer Learning
Fine Tuning and Feature Extraction
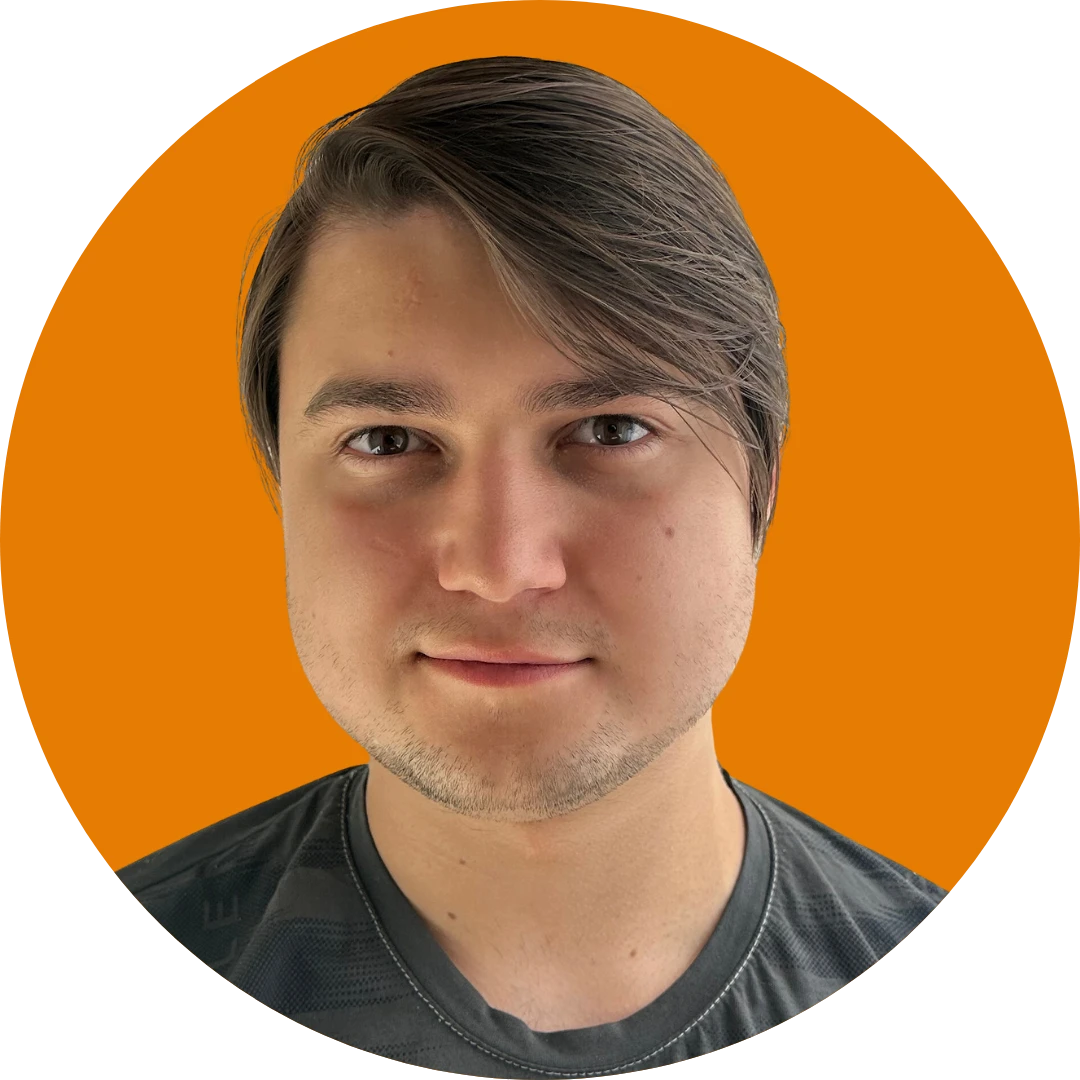
by Andrii Chornyi
Data Scientist, ML Engineer
Dec, 2023・9 min read
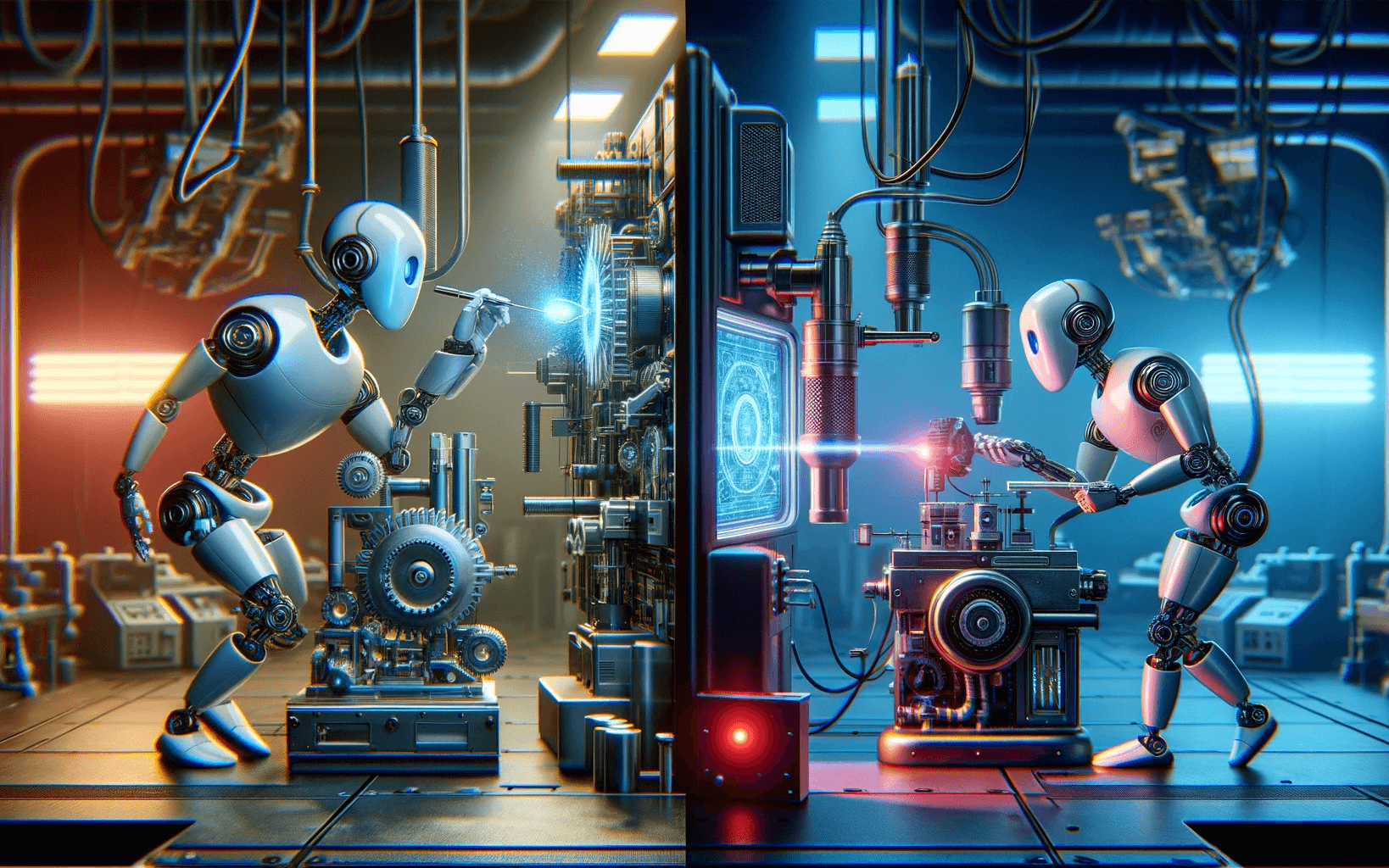
Supervised vs Unsupervised Learning
Supervised vs Unsupervised Learning
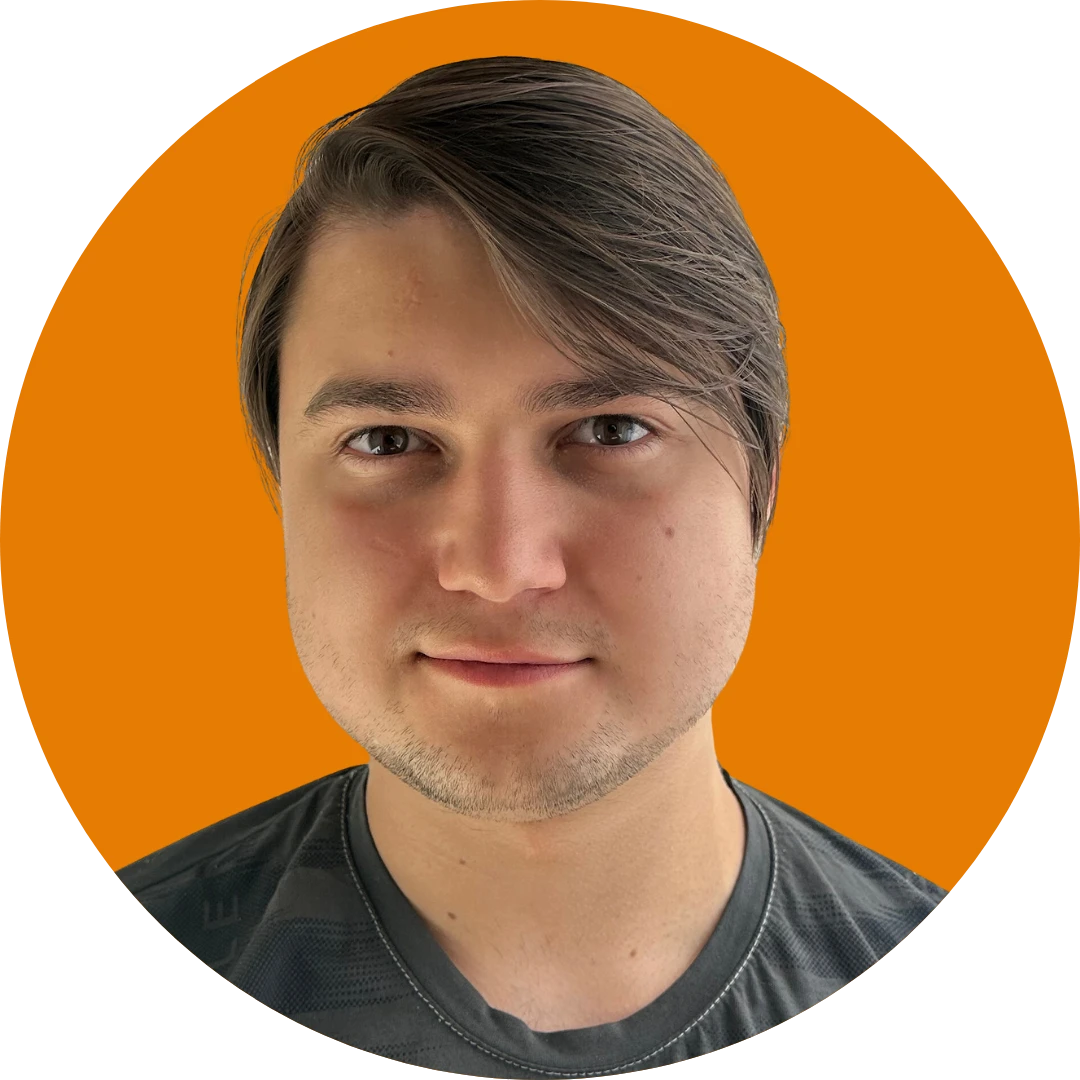
by Andrii Chornyi
Data Scientist, ML Engineer
Dec, 2023・7 min read
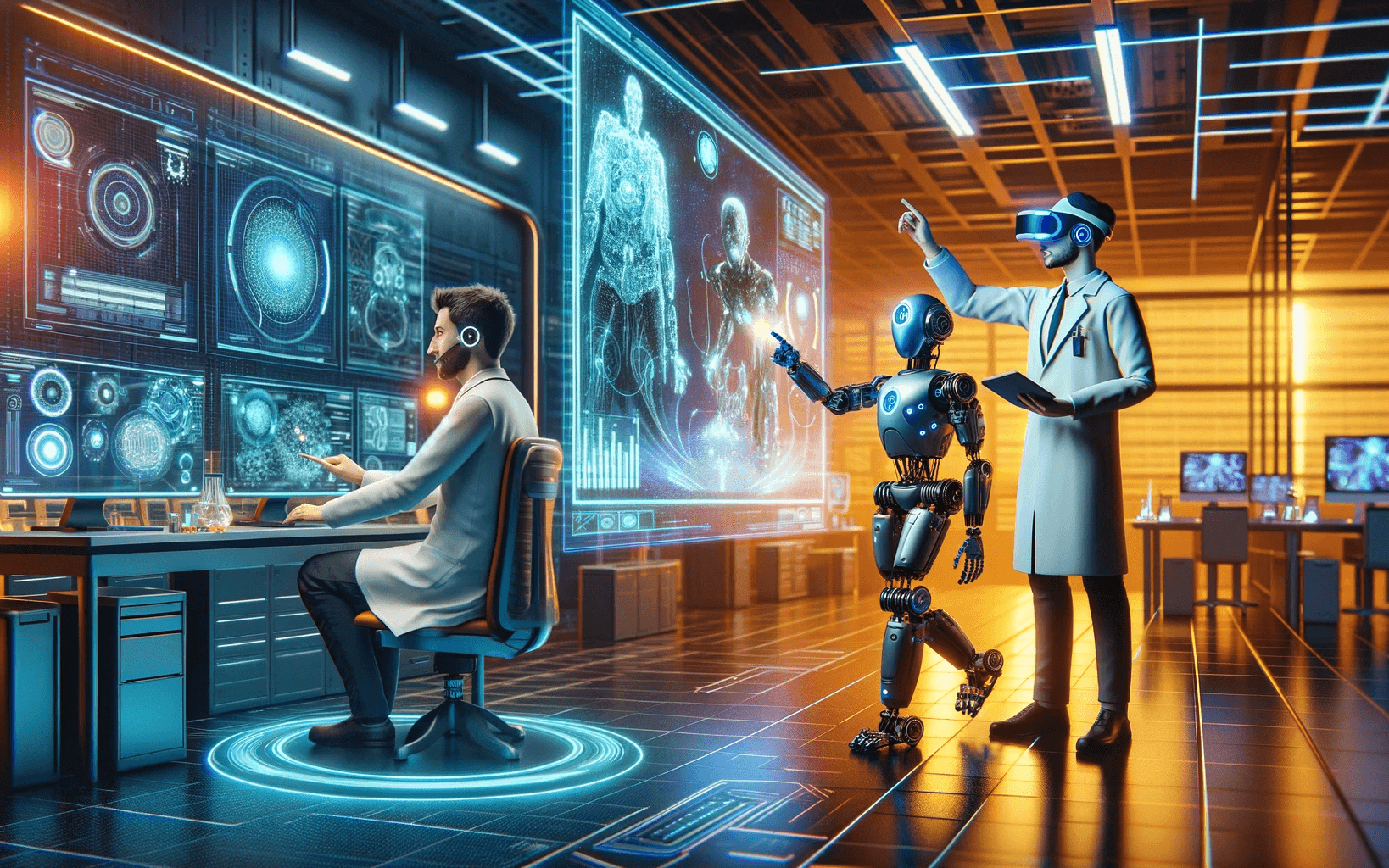
Conteúdo deste artigo