Курси по темі
Всі курсиБазовий
Introduction to Python
Python is a high-level, interpreted, general-purpose programming language. Distinguished from languages such as HTML, CSS, and JavaScript, which are mainly utilized in web development, Python boasts versatility across multiple domains, including software development, data science, and back-end development. This course will guide you through Python's fundamental concepts, equipping you with the skills to create your own functions by the conclusion of the program.
Середній
Intermediate Python Techniques
In this course, you will learn advanced topics such as function arguments, iterators, generators, closures, and decorators. Dozens of practice exercises will help you grasp these topics.
A Comprehensive Guide to Sentiment Analysis with Python
Unlocking Emotions in Text
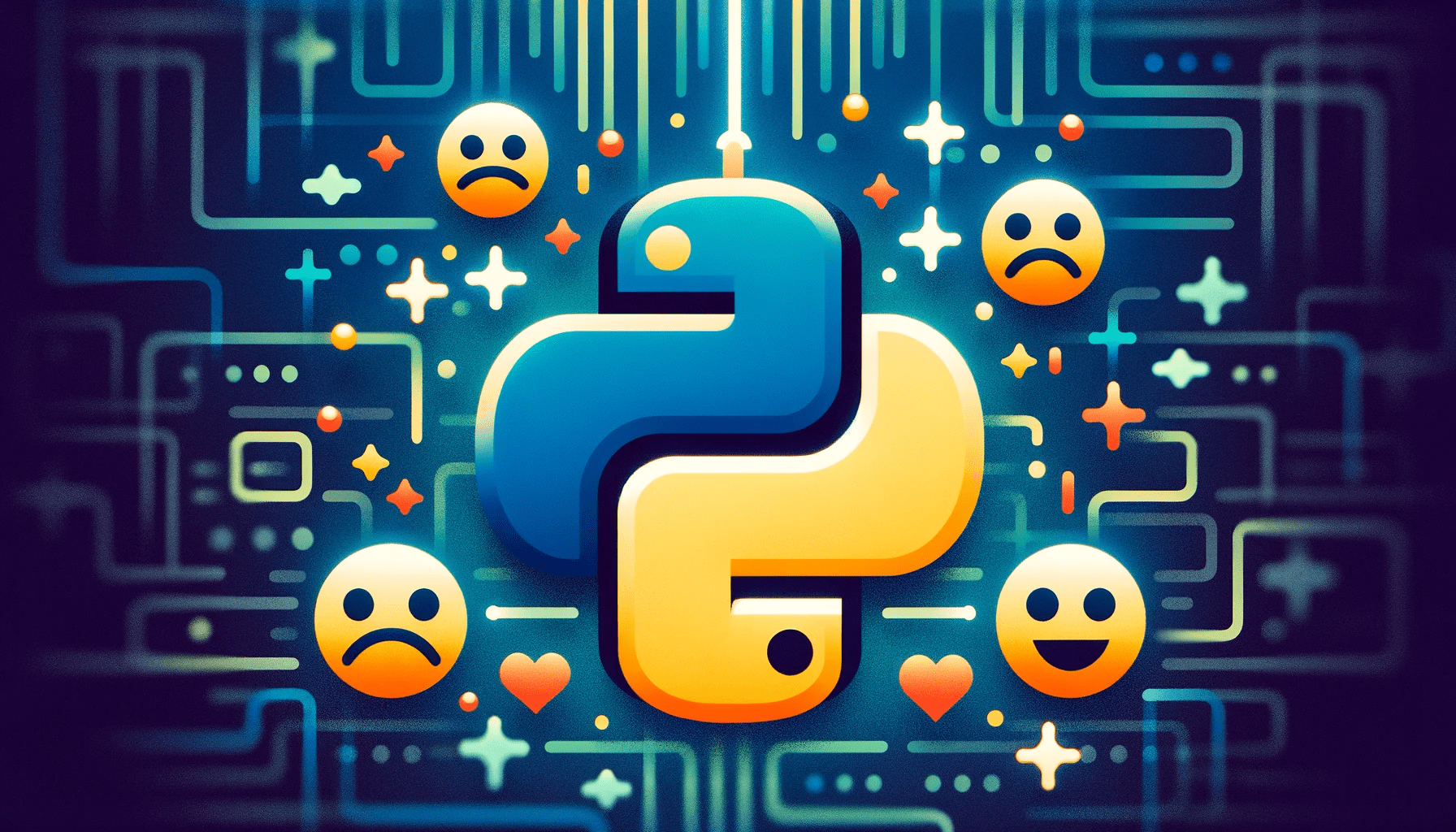
Sentiment analysis is a specialized area within natural language processing (NLP) which offers fascinating insights into the emotional undertones of textual data. This comprehensive guide delves into sentiment analysis using Python, exploring essential concepts, methodologies, and practical applications.
What is Sentiment Analysis?
Sentiment analysis, also known as opinion mining, involves identifying and categorizing opinions expressed in text in order to understand the sentiments embedded within it. This technique is pivotal in various fields, including marketing, customer service, and social media monitoring, as it helps in gauging public sentiment towards products, services, or ideas. It's particularly relevant in today's digital era where vast amounts of text data are generated daily.
Why Python for Sentiment Analysis?
Python emerges as a prime choice for sentiment analysis for several reasons:
- Rich Library Ecosystem: Python's extensive libraries like NLTK, TextBlob, and scikit-learn provide specialized tools for NLP, making it easier to process and analyze text data.
- Simplicity and Flexibility: Python's syntax is clear and readable, making it accessible to beginners. It also offers flexibility in solving complex NLP tasks.
- Strong Community Support: Python has a vast community of developers and data scientists, ensuring abundant resources and support for learners.
Run Code from Your Browser - No Installation Required
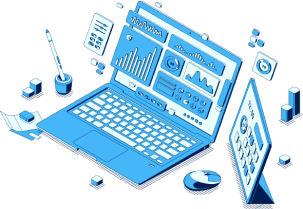
Getting Started with Python for Sentiment Analysis
First and foremost, ensure Python is installed on your machine. If not, you can install it by following along The beginner's guide: How to Install Python, SQL, and R. After installation, you can use Python's package manager, pip, to install all the necessary libraries:
python
Let's explore each of them in more detail:
- NLTK (Natural Language Toolkit): This library is a powerhouse for natural language processing, offering modules for classification, tokenization, stemming, tagging, parsing, and more. Its comprehensive set of tools makes it ideal for a wide range of NLP tasks.
- TextBlob: TextBlob simplifies text processing. It offers an intuitive API for tasks like part-of-speech tagging, noun phrase extraction, and sentiment analysis, making it a great starting point for beginners.
- Scikit-learn: Known for its machine learning capabilities, scikit-learn is used for developing complex models, including those for sentiment analysis. It provides a wide array of algorithms and tools for data preprocessing, classification, regression, and clustering.
Understanding Text Processing
Effective sentiment analysis begins with proper text processing:
- Tokenization: This process involves breaking text down into smaller units, like words or sentences. It's a crucial step in understanding the context and structure of the text.
- Stop Words Removal: Stop words are common words like "is", "and", "the", which are often removed since they contribute little to the overall meaning of the text.
- Stemming and Lemmatization: These techniques reduce words to their base or root form. Stemming chops off word endings, while lemmatization considers the context to convert a word to its base form.
Sentiment Analysis Techniques
- Rule-Based Systems: These systems utilize a set of predefined rules and lexicons (lists of words and their associated sentiments). The sentiment of a text is determined based on the presence and combination of these words.
- Automatic Systems: These leverage machine learning algorithms to learn sentiment from data. They require a dataset of texts with pre-labeled sentiments to train models.
- Hybrid Systems: Combining rule-based and automatic systems can sometimes yield better accuracy by leveraging the strengths of both approaches.
Start Learning Coding today and boost your Career Potential
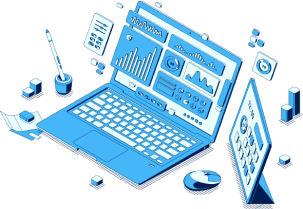
Practical Application: Analyzing Movie Reviews
To better understand sentiment analysis, let's apply it to a dataset of movie reviews.
Dataset: We will use a publicly available dataset of movie reviews from IMDb.
Step 1: Data Preparation
Begin by loading and preprocessing your dataset:
python
Step 2: Sentiment Analysis
We'll use TextBlob for a simple sentiment analysis:
python
Step 3: Results Interpretation
The polarity scores, which range from -1 (very negative) to 1 (very positive), help us gauge the general sentiment of each review. Analyzing these scores can provide insights into public opinion about different movies.
FAQs
Q: Do I need prior programming experience to learn sentiment analysis in Python?
A: While basic Python knowledge is beneficial, sentiment analysis can be approached as a beginner with the right resources and learning path.
Q: What are the common challenges in sentiment analysis?
A: Sentiment analysis can be complicated by factors like sarcasm, idioms, and context-dependent meanings, which are hard for algorithms to accurately interpret.
Q: Can sentiment analysis be used in languages other than English?
A: Yes. However, it requires language-specific resources, like sentiment lexicons and pre-trained models, which are not as readily available as for English.
Q: How accurate is sentiment analysis?
A: The accuracy depends on several factors: the quality of the dataset, the complexity of the text, and the sophistication of the model used. Generally, simpler texts yield more accurate results.
Q: Are there any ethical concerns with sentiment analysis?
A: Yes. Privacy and bias are significant concerns in sentiment analysis. It's important to consider the ethical implications of analyzing user-generated content and to use these tools responsibly.
Курси по темі
Всі курсиБазовий
Introduction to Python
Python is a high-level, interpreted, general-purpose programming language. Distinguished from languages such as HTML, CSS, and JavaScript, which are mainly utilized in web development, Python boasts versatility across multiple domains, including software development, data science, and back-end development. This course will guide you through Python's fundamental concepts, equipping you with the skills to create your own functions by the conclusion of the program.
Середній
Intermediate Python Techniques
In this course, you will learn advanced topics such as function arguments, iterators, generators, closures, and decorators. Dozens of practice exercises will help you grasp these topics.
Data Analyst vs Data Engineer vs Data Scientist
Unraveling the Roles and Responsibilities in Data-Driven Careers
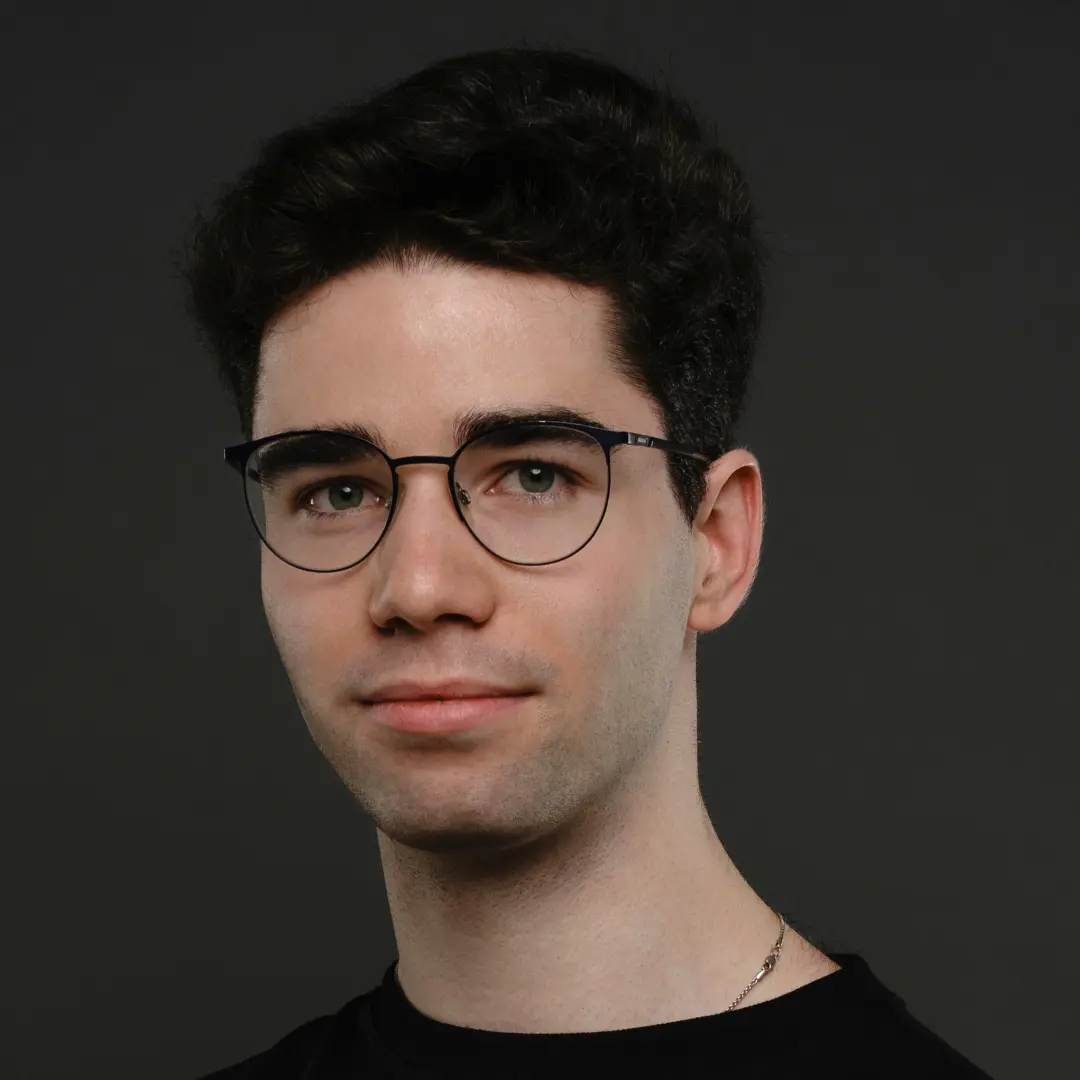
by Kyryl Sidak
Data Scientist, ML Engineer
Dec, 2023・7 min read
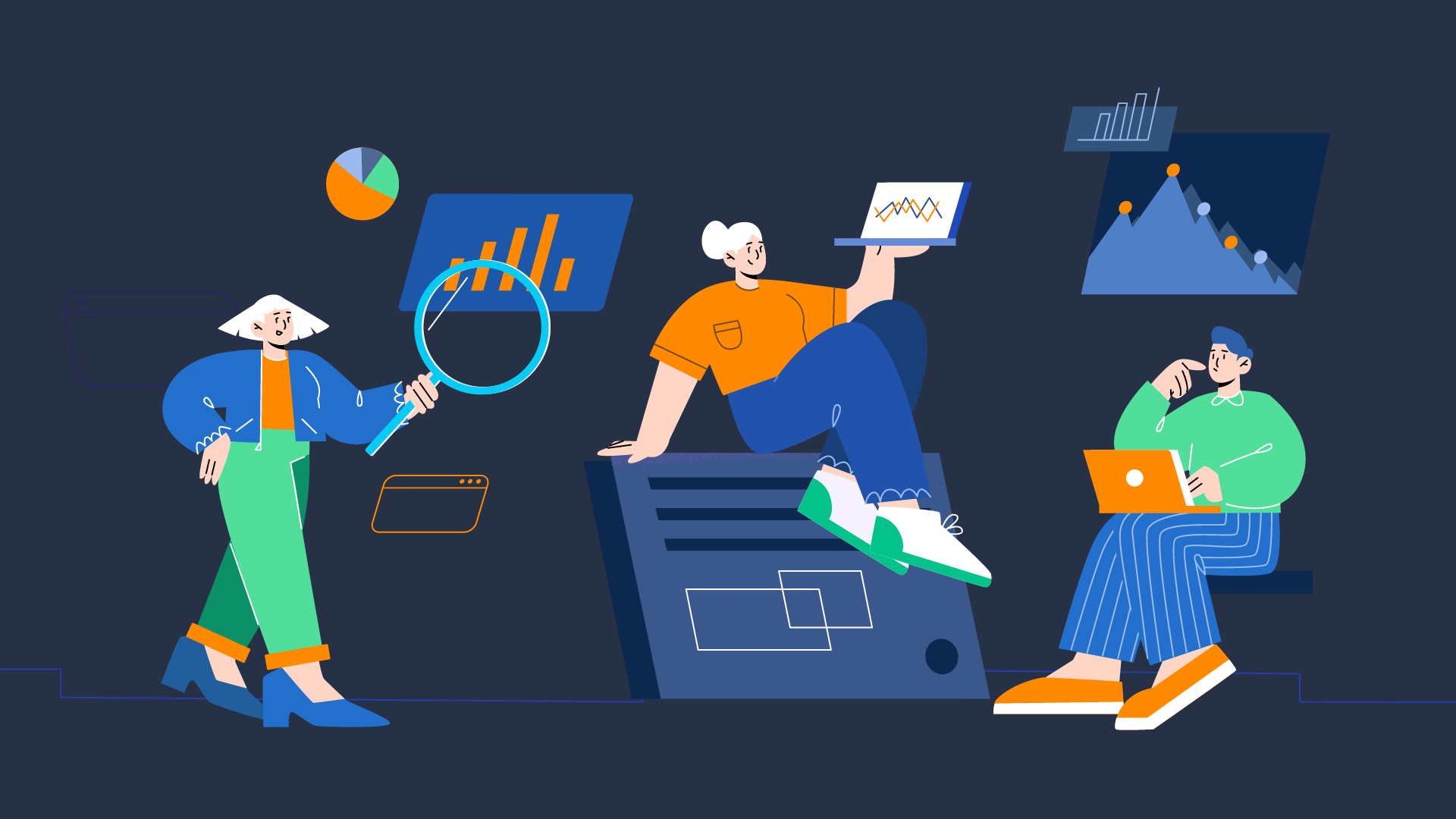
Explainable AI - Why It Matters and How It Works
From Confusion to Clarity: Making AI Decisions Transparent and Accountable
by Arsenii Drobotenko
Data Scientist, Ml Engineer
Jun, 2025・10 min read
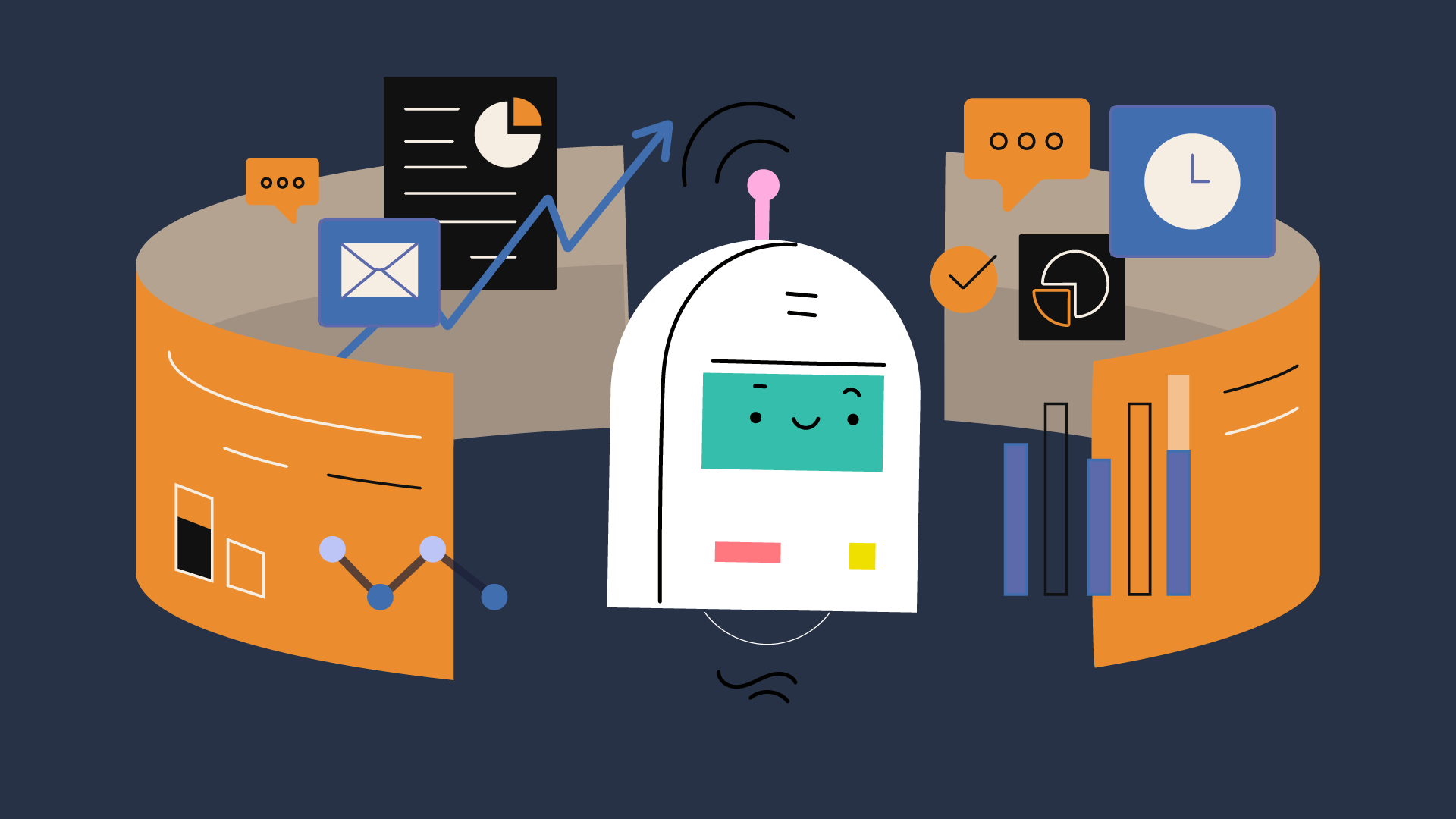
Top 3 SQL Certifications
How to Confirm Your SQL Skills
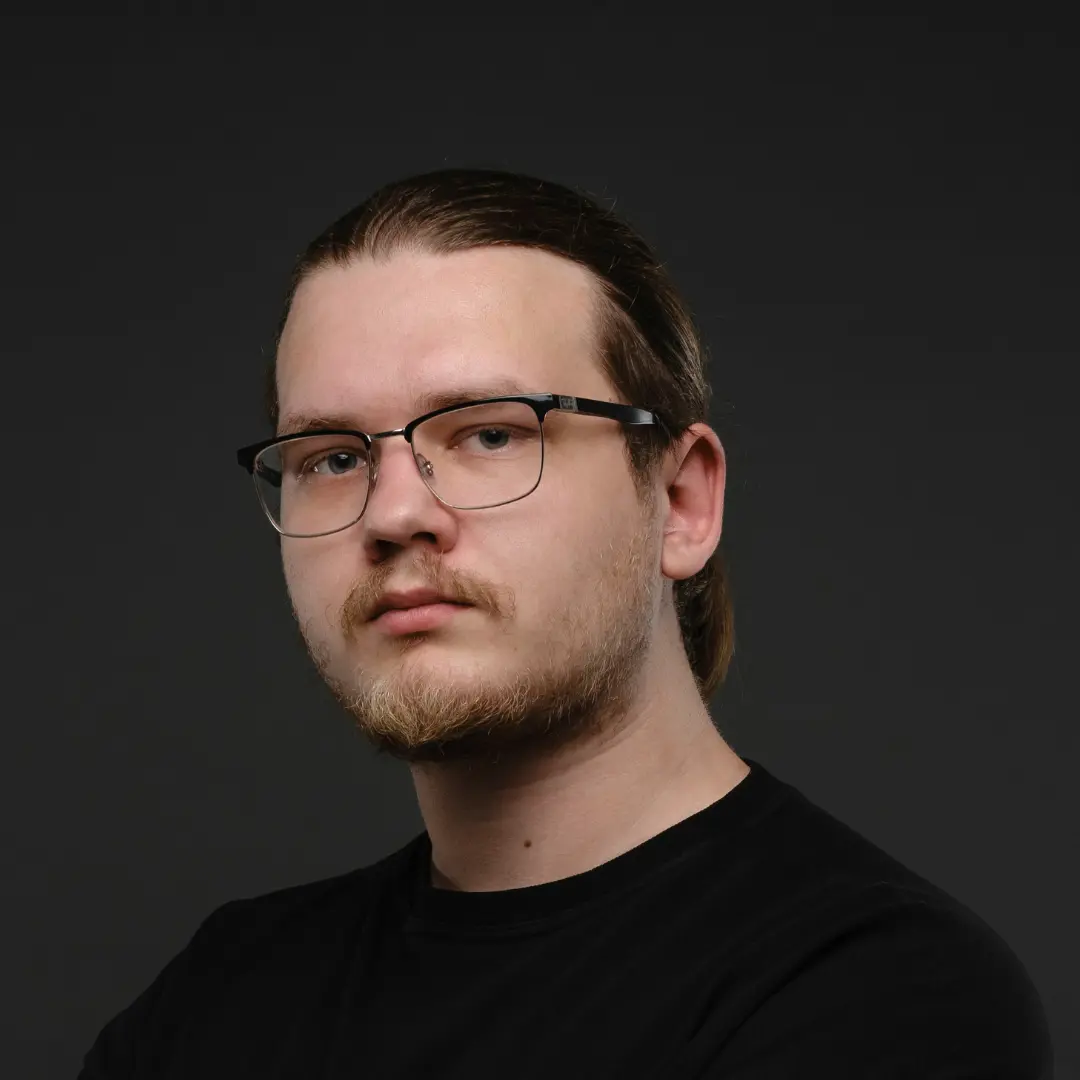
by Daniil Lypenets
Full Stack Developer
Sep, 2023・9 min read
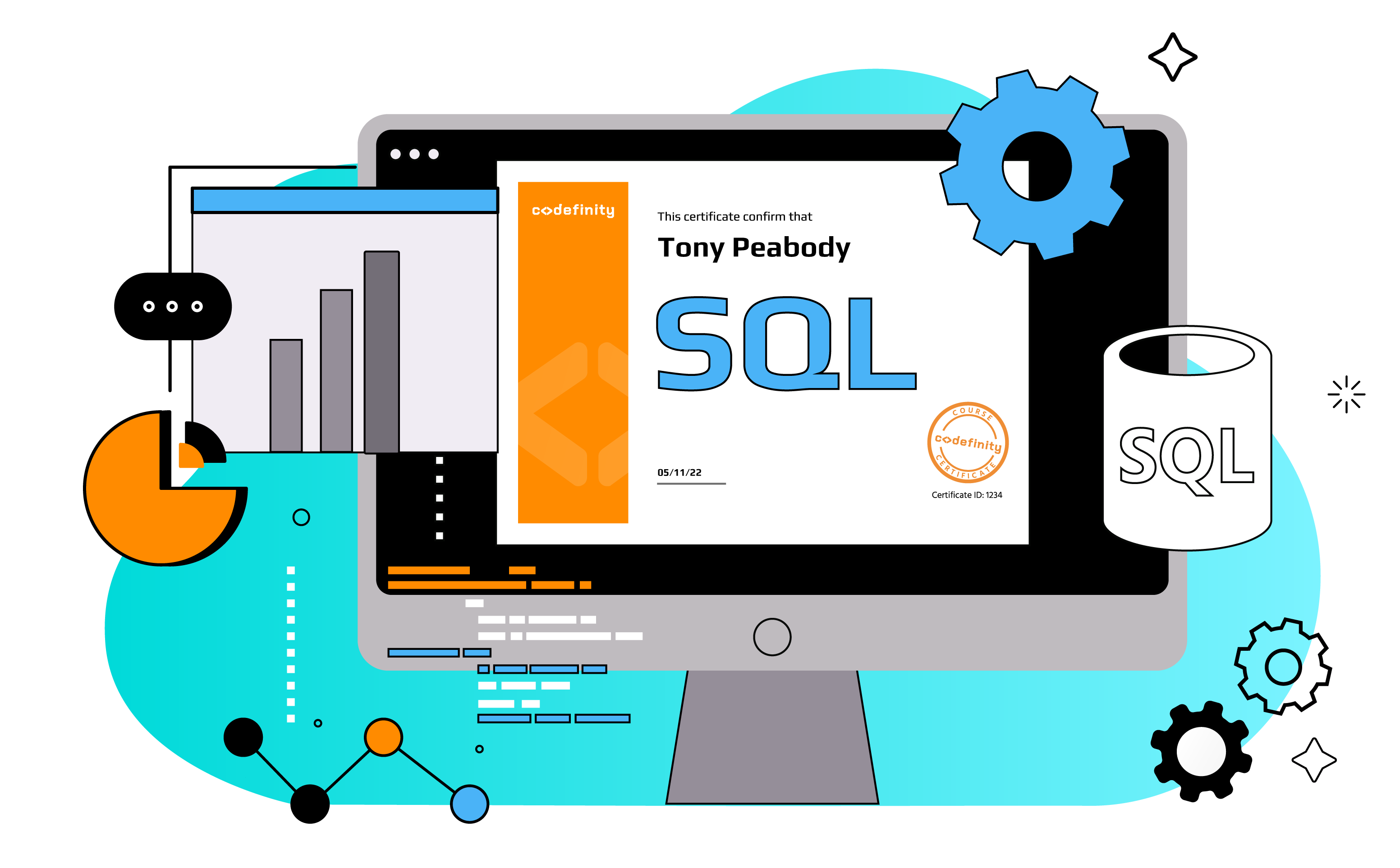
Зміст