Related courses
See All CoursesIntermediate
Time Series Analysis
What can be done with thousands of online store purchase records? How can we analyze this data and predict its growth? In this course, you will learn what parameters we can analyze in time series and how to create predictive models. Let's get started!
Intermediate
Probability Theory Basics
Probability theory is a fundamental branch of mathematics that deals with the study of uncertainty and randomness. It provides a framework for understanding and quantifying uncertainty in various fields, including statistics, data analysis, machine learning, finance, physics, and more.
Advanced
Advanced Probability Theory
Statistics and probability theory are fundamental tools in data analysis, decision-making, and scientific research. They provide a systematic and quantitative way to understand and interpret data, make predictions, and draw conclusions based on evidence. Now we will consider all additional topics necessary for Data Science and Data Analytics.
What is Econometrics
Intro to Econometrics

Introduction
Econometrics is a fascinating field at the intersection of economics, mathematics, and statistics. It plays a pivotal role in understanding, analyzing, and modeling complex economic phenomena. By applying rigorous quantitative methods to economic data, econometrics allows us to unravel the intricate relationships between economic variables and make informed predictions about economic outcomes.
Run Code from Your Browser - No Installation Required
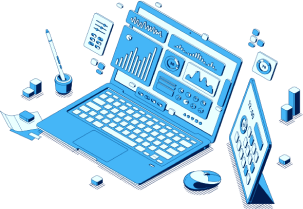
Econometric Models
Econometric models are the cornerstone of econometrics, enabling economists and analysts to quantitatively study and understand economic relationships. These models provide a structured framework for examining how various economic variables interact and influence each other. Here, we delve into the key aspects of econometric models:
What Are Econometric Models?
At their core, econometric models are mathematical representations of economic phenomena. They seek to capture the functional relationships between different economic variables, allowing researchers to make predictions, test hypotheses, and derive insights.
Types of Econometric Models
Econometric models can take several forms, including:
-
Linear Regression Models: These models assume a linear relationship between the dependent variable and one or more independent variables. They are commonly used for analyzing cross-sectional data.
-
Time Series Models: Time series econometric models are designed to analyze data collected over time, such as stock prices, GDP growth, or monthly sales figures. Examples include autoregressive integrated moving average (ARIMA) models.
-
Structural Models: Structural econometric models incorporate economic theory to establish causal relationships. They are often used to study the impact of policy changes or economic shocks.
-
Simultaneous Equation Models: These models involve a system of equations where variables are jointly determined. They are used to explore interactions among multiple economic variables.
Model Specification
Model specification involves defining the functional form of the model, selecting relevant variables, and specifying the relationships between them. This step is crucial as it sets the foundation for empirical analysis.
Estimation Techniques
Estimation is the process of obtaining numerical values for the model's parameters based on available data. Common estimation techniques in econometrics include:
-
Ordinary Least Squares (OLS): OLS is a widely used method for estimating parameters in linear regression models.
-
Maximum Likelihood Estimation (MLE): MLE is used for estimating parameters when the likelihood function is known.
-
Instrumental Variables (IV): IV methods are employed to address endogeneity issues by finding suitable instruments that are correlated with the endogenous variables.
Model Testing and Validation
Once a model is estimated, it must undergo rigorous testing and validation to ensure its validity. This includes assessing model fit, examining the residuals for statistical properties, and conducting hypothesis tests.
Interpretation and Policy Implications
Interpreting econometric models involves analyzing the estimated coefficients and their economic significance. These interpretations guide decision-making and inform policymakers about the implications of various economic scenarios.
Econometric models are indispensable tools for economists, researchers, and policymakers, allowing them to gain insights into economic phenomena, make informed decisions, and develop effective policies.
Econometrics vs Machine Learning
Aspect | Econometrics | Machine Learning |
---|---|---|
Objective | Analyze and model economic relationships and | Predict outcomes or classify data |
test economic theories. | based on patterns and algorithms. | |
Data Requirements | Typically relies on structured and | Can work with structured, |
domain-specific data. | semi-structured, or unstructured data. | |
Model Complexity | Often employs simpler, interpretable models | Utilizes complex algorithms and deep |
such as linear regression. | neural networks. | |
Assumptions | Relies on economic assumptions and | Assumptions may vary but are often |
theoretical foundations. | data-driven and less theoretical. | |
Causality | Focuses on identifying causal relationships | Emphasizes prediction, making causal |
and understanding direction of effects. | inference challenging. | |
Interpretability | Provides easily interpretable coefficients | Models may lack interpretability due |
and economic insights. | to complexity. | |
Feature Engineering | Typically uses domain knowledge for feature | Often involves automated feature |
selection and engineering. | extraction and selection. | |
Model Validation | Employs statistical tests and validation | Relies on cross-validation, metrics, |
techniques tailored to econometric models. | and ensemble methods for validation. | |
Domain Expertise | Requires economic expertise for model | Less reliant on domain expertise and |
specification and interpretation. | more on data and algorithms. | |
Time Series Analysis | Commonly used for time-dependent data | Applies to a wide range of data |
and forecasting. | types, including time series. | |
Ethical Considerations | Addresses ethical issues in economics, | Ethical concerns revolve around data |
such as bias and fairness. | privacy and algorithmic bias. | |
Applications | Widely used in economics, finance, healthcare, | Applied in various domains including |
and social sciences. | healthcare, finance, and robotics. |
Start Learning Coding today and boost your Career Potential
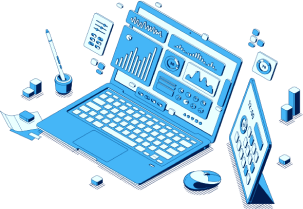
FAQs
Q: What is the primary goal of econometrics?
A: The primary goal of econometrics is to apply statistical and mathematical methods to economic data in order to quantify and understand the relationships between economic variables and make informed predictions.
Q: What are some common econometric models?
A: Common econometric models include linear regression, time series models, panel data models, and structural equation models, among others, depending on the specific research or analysis objectives.
Q: How does econometrics differ from statistics?
A: While both fields involve statistical techniques, econometrics is specifically tailored to economic data and focuses on addressing economic questions, hypotheses, and theories using statistical methods.
Q: What types of data are typically used in econometrics?
A: Econometrics utilizes various types of data, including time-series data (over time), cross-sectional data (across different entities), and panel data (combining both time and entities) to analyze economic relationships.
Q: What role does causality play in econometrics?
A: Causality is a fundamental concept in econometrics, as it seeks to establish causal relationships between economic variables and understand the direction of cause and effect in economic phenomena.
Q: Can econometric models be used for policy analysis?
A: Yes, econometric models are commonly used for policy analysis to assess the potential impact of policy changes on economic outcomes and make informed policy recommendations.
Q: What skills are important for someone interested in econometrics?
A: Proficiency in statistics, mathematics, data analysis, and economic theory is crucial for those interested in econometrics. Strong analytical and programming skills are also valuable.
Q: How is econometrics applied in real-world scenarios?
A: Econometrics is applied in various real-world scenarios, including economic forecasting, financial modeling, impact evaluations of government policies, and market research.
Q: What are some ethical considerations in econometrics?
A: Ethical considerations in econometrics include ensuring data privacy, addressing bias and discrimination, and transparently reporting results to avoid misinterpretation or misuse.
Q: What are the future trends in econometrics?
A: The future of econometrics may involve the integration of machine learning techniques, big data analysis, and advancements in causal inference methods to address complex economic questions.
Related courses
See All CoursesIntermediate
Time Series Analysis
What can be done with thousands of online store purchase records? How can we analyze this data and predict its growth? In this course, you will learn what parameters we can analyze in time series and how to create predictive models. Let's get started!
Intermediate
Probability Theory Basics
Probability theory is a fundamental branch of mathematics that deals with the study of uncertainty and randomness. It provides a framework for understanding and quantifying uncertainty in various fields, including statistics, data analysis, machine learning, finance, physics, and more.
Advanced
Advanced Probability Theory
Statistics and probability theory are fundamental tools in data analysis, decision-making, and scientific research. They provide a systematic and quantitative way to understand and interpret data, make predictions, and draw conclusions based on evidence. Now we will consider all additional topics necessary for Data Science and Data Analytics.
Data Analyst vs Data Engineer vs Data Scientist
Unraveling the Roles and Responsibilities in Data-Driven Careers
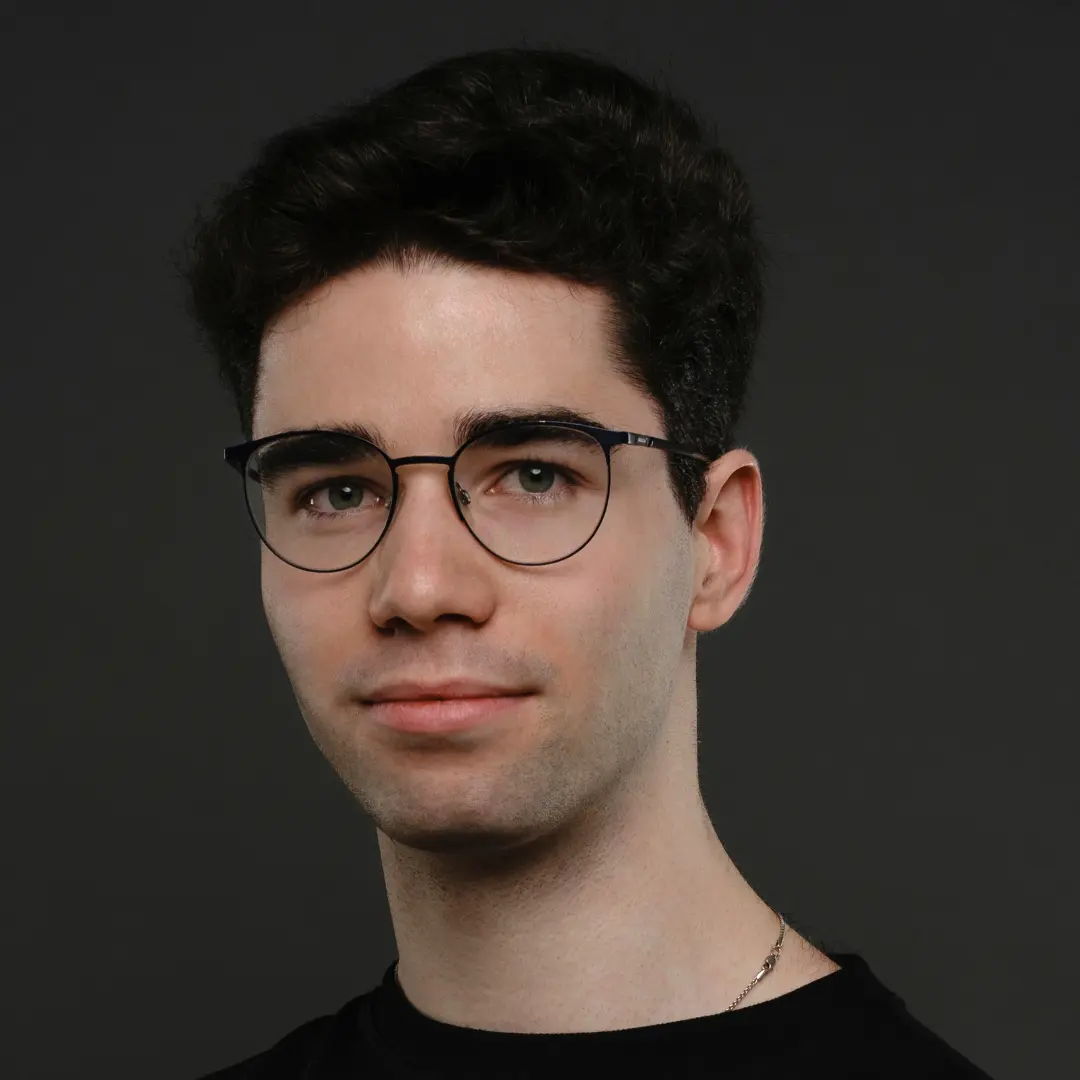
by Kyryl Sidak
Data Scientist, ML Engineer
Dec, 2023・7 min read
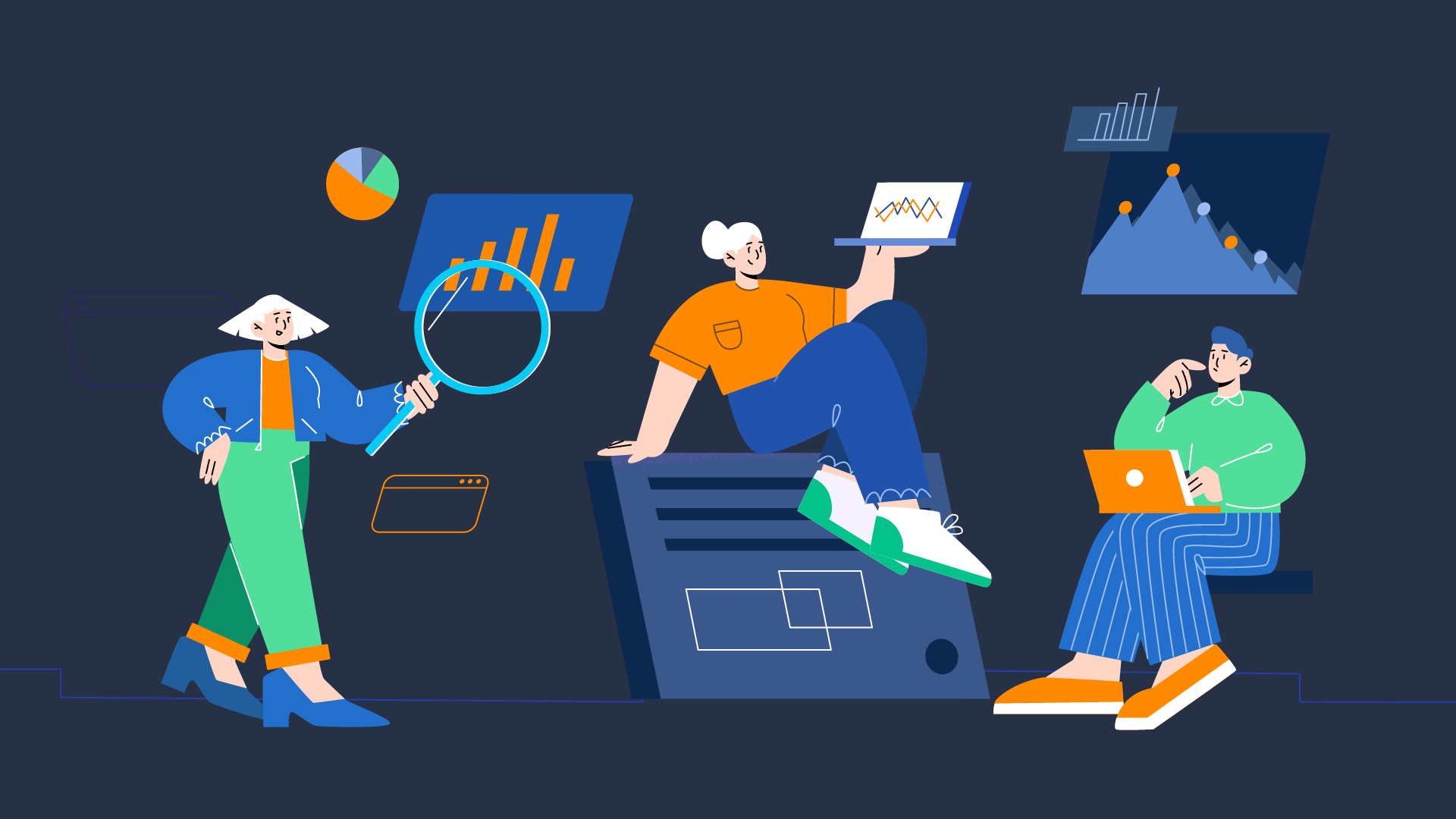
Top 3 SQL Certifications
How to Confirm Your SQL Skills
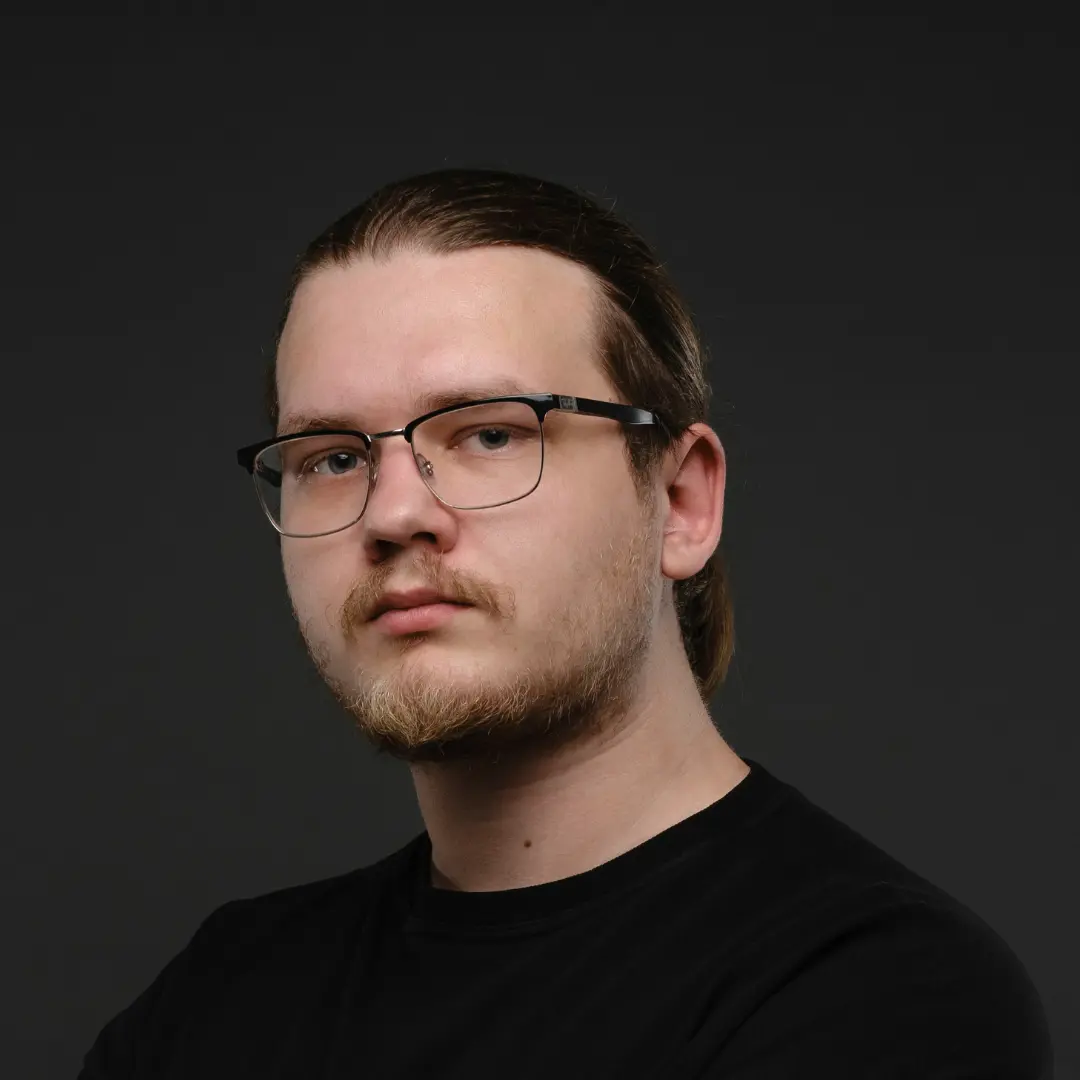
by Daniil Lypenets
Full Stack Developer
Sep, 2023・9 min read
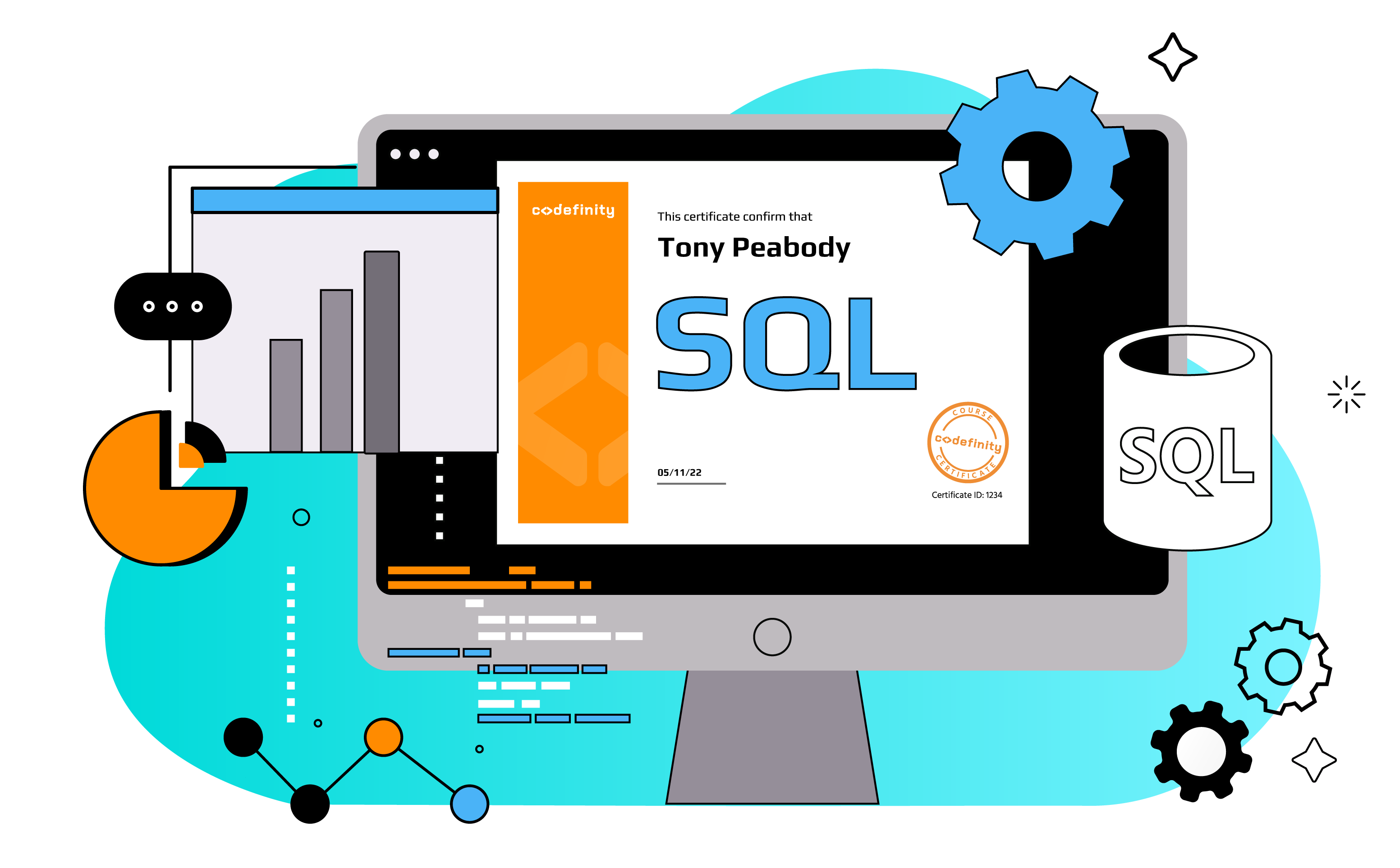
Understanding Cognitive Distortions in Data Analytics
Top cognitive distortions in Data Analytics
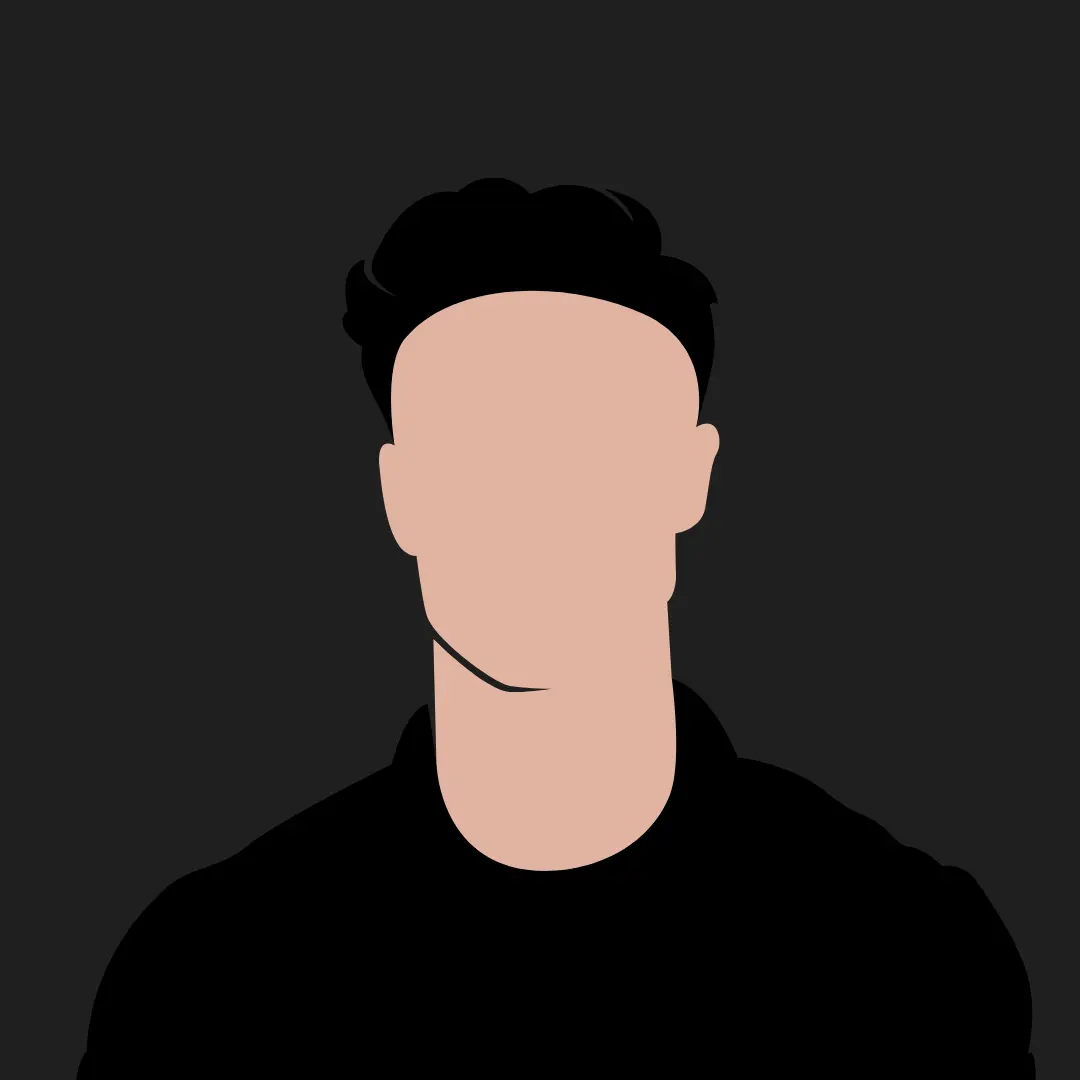
by Ruslan Shudra
Data Scientist
Dec, 2023・14 min read
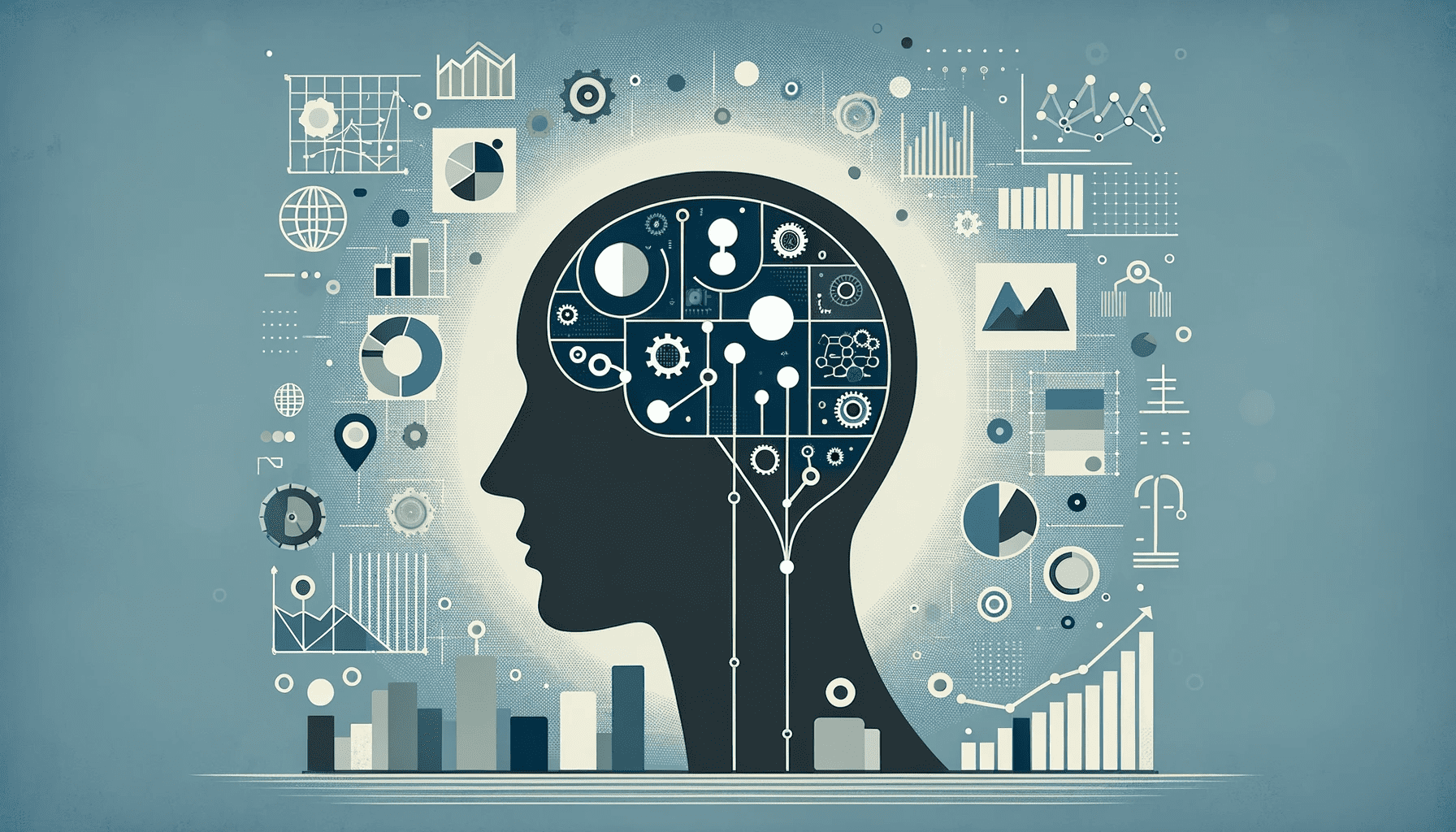
Content of this article