Related courses
See All CoursesIntermediate
Mathematics for Data Analysis and Modeling
To study many applied disciplines, it is necessary to have basic knowledge of higher mathematics and linear algebra. Mathematics is often used in data analysis, system modeling, building machine learning models, etc. Let's look at some of the most important topics and learn how to use the mathematical apparatus to solve real problems.
Intermediate
Probability Theory Basics
Probability theory is a fundamental branch of mathematics that deals with the study of uncertainty and randomness. It provides a framework for understanding and quantifying uncertainty in various fields, including statistics, data analysis, machine learning, finance, physics, and more.
Advanced
Advanced Probability Theory
Statistics and probability theory are fundamental tools in data analysis, decision-making, and scientific research. They provide a systematic and quantitative way to understand and interpret data, make predictions, and draw conclusions based on evidence. Now we will consider all additional topics necessary for Data Science and Data Analytics.
Understanding Cognitive Distortions in Data Analytics
Top cognitive distortions in Data Analytics
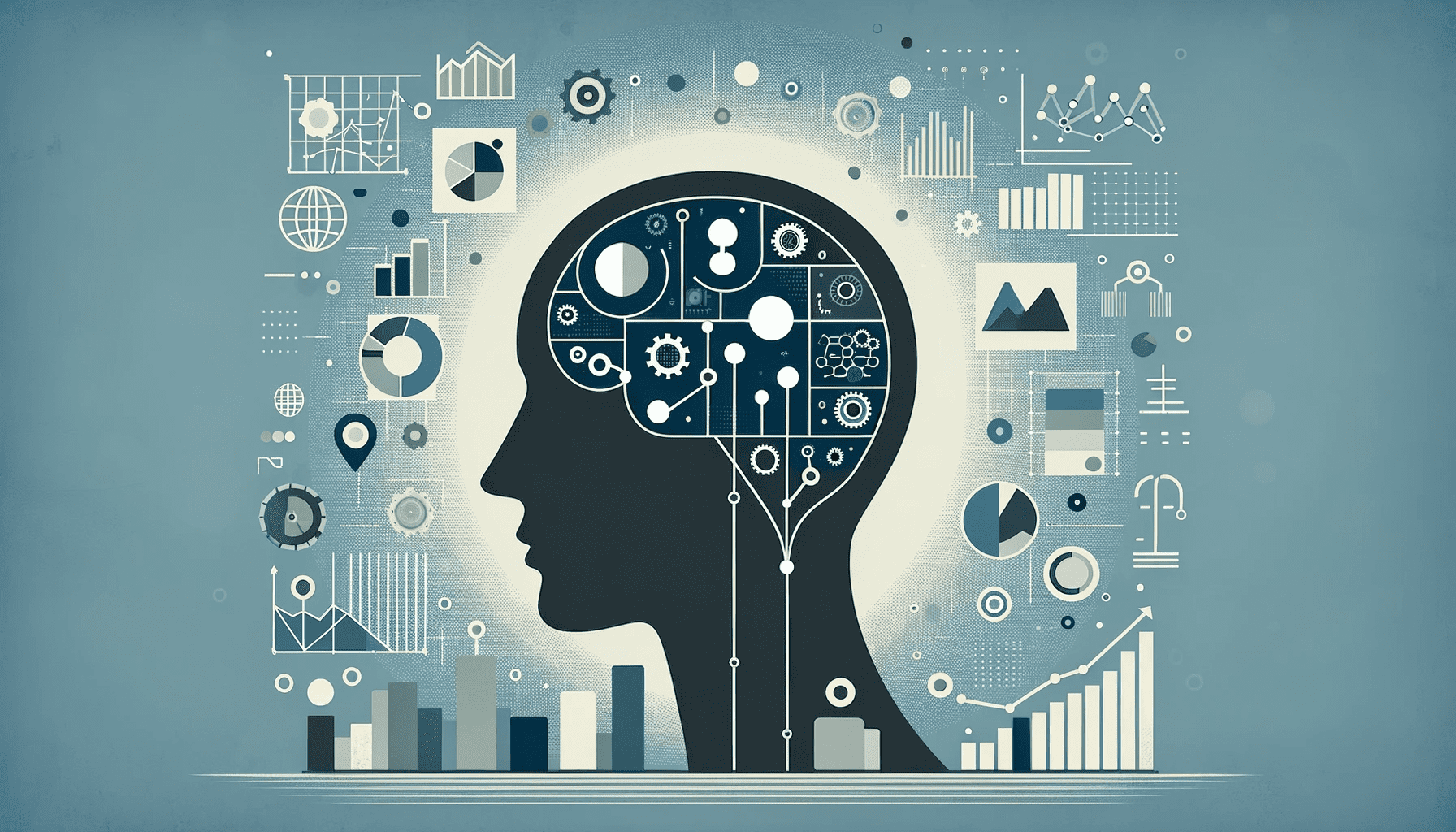
Introduction
In the world of data analytics, making informed decisions is paramount. Data-driven insights fuel business strategies, guide critical choices, and influence outcomes. However, even in a data-rich environment, human cognition can introduce biases and distortions that impact decision-making processes.
This article delves into the fascinating realm of cognitive distortions and their relevance in the field of data analytics. We'll explore seven cognitive biases that data analysts and decision-makers should be aware of to ensure more accurate, objective, and effective data-driven decisions.
By understanding these cognitive distortions and learning how to mitigate their effects, data professionals can enhance the quality of their analyses, avoid common pitfalls, and harness the true power of data for informed decision-making. Let's embark on this journey to uncover the nuances of cognitive biases in data analytics.
Confirmation Bias
Confirmation bias is a cognitive bias that influences the way individuals gather and interpret information. It refers to the tendency to search for, favor, and recall information that confirms or supports one's existing beliefs, hypotheses, or preconceptions, while selectively ignoring or downplaying contradictory evidence.
How Confirmation Bias Operates
-
Information Selection: People with confirmation bias often actively seek out information that aligns with their preexisting views. This can lead to a limited exposure to diverse perspectives and data.
-
Interpretation: When faced with ambiguous or mixed evidence, individuals tend to interpret it in a way that reinforces their existing beliefs, thereby reinforcing their initial convictions.
-
Recall: Confirmation bias can also influence memory. People are more likely to remember information that confirms their beliefs and forget or dismiss information that contradicts them.
Impact on Data Analytics
In the context of data analytics, confirmation bias can significantly impact decision-making and analysis in several ways:
-
Selective Data Collection: Analysts may unintentionally collect data that supports their initial assumptions, ignoring potentially valuable and contradictory data sources.
-
Interpretation: Data analysts may interpret data in a manner that aligns with their hypotheses or expectations, potentially leading to biased conclusions.
-
Modeling and Prediction: Confirmation bias can affect the development of predictive models, leading to overfitting when models are overly influenced by existing beliefs.
-
Reporting and Communication: The bias can manifest in the way data findings are presented to stakeholders, emphasizing positive results while downplaying challenges or limitations.
Mitigating Confirmation Bias
To mitigate confirmation bias in data analytics, it's essential to:
- Foster a culture of openness to diverse viewpoints and data sources.
- Encourage critical thinking and self-awareness among data analysts.
- Employ blind or double-blind analysis techniques where possible.
- Implement rigorous peer review processes to challenge assumptions.
- Conduct sensitivity analyses to assess the impact of different interpretations.
By recognizing and actively addressing confirmation bias, data analysts can enhance the objectivity and reliability of their analyses, leading to more accurate and valuable insights.
Run Code from Your Browser - No Installation Required
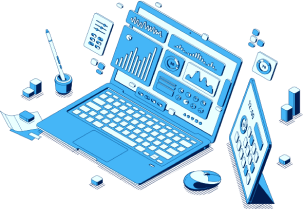
Overconfidence Bias
Overconfidence bias, often referred to as the "overconfidence effect," is a cognitive bias that occurs when individuals overestimate their own knowledge, abilities, or the accuracy of their predictions. This bias can have a significant impact on decision-making processes in data analytics and other fields.
Characteristics of Overconfidence Bias:
-
Overestimation of Knowledge: Individuals affected by overconfidence bias tend to believe that they possess more knowledge and expertise than they actually do. This can lead to unwarranted confidence in their analytical skills and conclusions.
-
Overestimation of Predictions: People with overconfidence bias may overestimate the accuracy of their predictions or forecasts. They may believe that their models or analyses are more precise than they truly are.
-
Underestimation of Uncertainty: Overconfident individuals often underestimate the inherent uncertainty associated with data and predictions. They may not adequately account for potential errors or variability in their analyses.
Impact on Data Analytics:
Overconfidence bias can have several implications for data analytics:
-
Flawed Assumptions: Analysts may make assumptions about data or models without sufficient validation, leading to flawed analyses and incorrect conclusions.
-
Risk of Overinvestment: Overconfidence can lead to overinvestment in a particular analytic approach or hypothesis, potentially resulting in wasted resources.
-
Lack of Objectivity: Overconfident analysts may dismiss alternative viewpoints or neglect to consider competing hypotheses, reducing the objectivity of their analyses.
Mitigating Overconfidence Bias:
Mitigating overconfidence bias requires awareness and deliberate efforts:
-
Encourage Critical Thinking: Promote a culture of critical thinking within data analytics teams, where individuals challenge their assumptions and the assumptions of others.
-
Validation and Calibration: Validate models and predictions rigorously and calibrate them against real-world outcomes to assess their accuracy.
-
Seek Diverse Perspectives: Encourage collaboration and seek input from colleagues with varying perspectives to reduce the risk of overconfidence.
-
Document Assumptions: Clearly document assumptions, methodologies, and uncertainties in data analyses to promote transparency and accountability.
Recognizing overconfidence bias and taking steps to mitigate its effects is crucial for data professionals to enhance the quality and reliability of their data-driven decision-making processes.
Groupthink
In the realm of decision-making, Groupthink is a phenomenon that can hinder the quality of choices made within a group or team. It refers to the tendency of a cohesive group to prioritize consensus and harmony over critical evaluation of alternatives and the pursuit of innovative solutions.
Key Characteristics of Groupthink:
-
Illusion of Invulnerability: Group members may develop an overconfidence in their decisions, believing that they are immune to errors or failures.
-
Collective Rationalization: When faced with dissenting opinions or conflicting information, group members tend to downplay or rationalize away contrary viewpoints.
-
Belief in Inherent Morality: The group may develop a sense of moral superiority, believing that their decisions are morally right, leading to ethical blind spots.
-
Stereotyping of Outsiders: Outsiders or dissenting individuals are often viewed as adversaries or enemies, preventing diverse perspectives from being considered.
-
Self-Censorship: Group members may withhold their doubts or objections to avoid conflict or maintain group cohesion.
-
Illusion of Unanimity: Silence is interpreted as agreement, creating a false consensus within the group.
-
Direct Pressure on Dissenters: Individuals who express opposing views may face direct pressure to conform, leading to self-censorship and conformity.
-
Mindguards: Some group members may act as "mindguards," shielding the group from dissenting information or viewpoints.
Implications in Decision-Making:
Groupthink can lead to flawed decision-making processes and outcomes, often characterized by:
- Incomplete consideration of alternatives.
- Overlooking potential risks and negative consequences.
- A failure to identify and address critical issues.
- Reduced creativity and innovation.
- Missed opportunities for better solutions.
Avoiding and Mitigating Groupthink:
To prevent or mitigate the effects of Groupthink, decision-making groups can take several measures, including:
- Encouraging open and constructive debate.
- Assigning a devil's advocate role to challenge the prevailing consensus.
- Seeking external input or diverse perspectives.
- Conducting independent assessments and reviews.
- Promoting a culture of critical thinking and diversity of thought.
In conclusion, Groupthink is a cognitive bias that can hinder effective decision-making within groups or teams. Being aware of its characteristics and taking proactive steps to mitigate its effects is crucial for fostering more robust, innovative, and well-informed decisions in any collaborative setting.
Availability Heuristic
The Availability Heuristic is a cognitive bias that influences decision-making by relying on the ease with which information or examples come to mind. This mental shortcut can lead individuals to overestimate the importance of readily available information, leading to potentially biased judgments and decisions.
How It Works
The Availability Heuristic operates on the principle that people tend to assess the frequency, likelihood, or importance of an event or concept based on how easily they can recall relevant instances from memory. If a particular event or idea is more vivid, recent, or memorable, it is more likely to be considered significant, even if it is statistically less probable.
Examples
-
Media Influence: News reports often focus on dramatic or sensational events, making them more memorable. As a result, individuals may overestimate the frequency of such events, leading to heightened perceptions of risk or danger.
-
Marketing Impact: Advertisers use the Availability Heuristic to their advantage by creating memorable and catchy slogans or jingles. Consumers are more likely to choose products or services associated with these easily remembered advertisements.
-
Health Decision-Making: Patients may base their health-related decisions on recent and easily recalled anecdotes or experiences, rather than relying on statistical probabilities or medical advice.
Implications in Data Analytics
In data analytics, the Availability Heuristic can lead to biased interpretations and decisions. Analysts may give undue weight to data that is more accessible, such as recent trends or prominent outliers, while overlooking less salient but equally relevant information. This bias can affect forecasting, risk assessment, and the evaluation of data-driven insights.
Mitigation
To mitigate the influence of the Availability Heuristic, data analysts should adopt a more systematic and objective approach to decision-making. This includes:
- Conducting comprehensive data analysis, considering the full range of available data.
- Employing statistical methods and probability assessments to make decisions based on data trends and patterns.
- Being aware of the potential impact of cognitive biases and actively seeking to minimize their effects in the decision-making process.
By recognizing the presence of the Availability Heuristic and taking steps to address it, data analysts can enhance the accuracy and reliability of their data-driven decisions.
Start Learning Coding today and boost your Career Potential
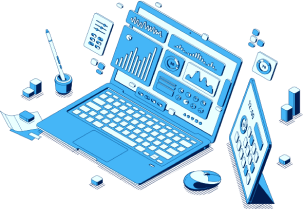
FAQs
Q: What are cognitive distortions in data analytics decision-making?
A: Cognitive distortions are systematic thinking errors or biases that can influence the process of making data-driven decisions. They can lead to skewed perceptions, misinterpretations of data, and suboptimal choices.
Q: How do cognitive distortions impact data analytics?
A: Cognitive distortions can affect data analytics by introducing bias, leading to incorrect conclusions, and influencing the interpretation of data. They may cause analysts to overemphasize certain data points, overlook relevant information, or misjudge the significance of findings.
Q: Why is it important to be aware of cognitive distortions in data analytics?
A: Awareness of cognitive distortions is crucial because it helps data professionals recognize and mitigate these biases. By understanding how cognitive distortions work, analysts can make more objective and accurate data-driven decisions.
Q: Are cognitive distortions prevalent only in data analytics, or do they occur in other fields as well?
A: Cognitive distortions are not unique to data analytics and can occur in various decision-making contexts. They are a part of human cognition and can affect decisions in areas such as psychology, economics, and everyday life.
Q: Can cognitive distortions be completely eliminated from the data analytics process?
A: Cognitive distortions cannot be entirely eliminated, as they are inherent in human thinking processes. However, data professionals can reduce their impact by being aware of them, employing systematic analysis methods, and seeking diverse perspectives.
Q: What are some common strategies for mitigating cognitive distortions in data analytics?
A: Mitigation strategies include conducting thorough data analysis, employing statistical methods, seeking input from diverse team members, and actively challenging cognitive biases during decision-making processes.
Q: How can I improve my data analytics skills while minimizing the impact of cognitive distortions?
A: Continuous learning, practice, and staying up-to-date with best practices in data analytics can help improve your skills. Additionally, fostering a culture of open dialogue and critical thinking within your team can contribute to better decision-making.
Related courses
See All CoursesIntermediate
Mathematics for Data Analysis and Modeling
To study many applied disciplines, it is necessary to have basic knowledge of higher mathematics and linear algebra. Mathematics is often used in data analysis, system modeling, building machine learning models, etc. Let's look at some of the most important topics and learn how to use the mathematical apparatus to solve real problems.
Intermediate
Probability Theory Basics
Probability theory is a fundamental branch of mathematics that deals with the study of uncertainty and randomness. It provides a framework for understanding and quantifying uncertainty in various fields, including statistics, data analysis, machine learning, finance, physics, and more.
Advanced
Advanced Probability Theory
Statistics and probability theory are fundamental tools in data analysis, decision-making, and scientific research. They provide a systematic and quantitative way to understand and interpret data, make predictions, and draw conclusions based on evidence. Now we will consider all additional topics necessary for Data Science and Data Analytics.
Data Analyst vs Data Engineer vs Data Scientist
Unraveling the Roles and Responsibilities in Data-Driven Careers
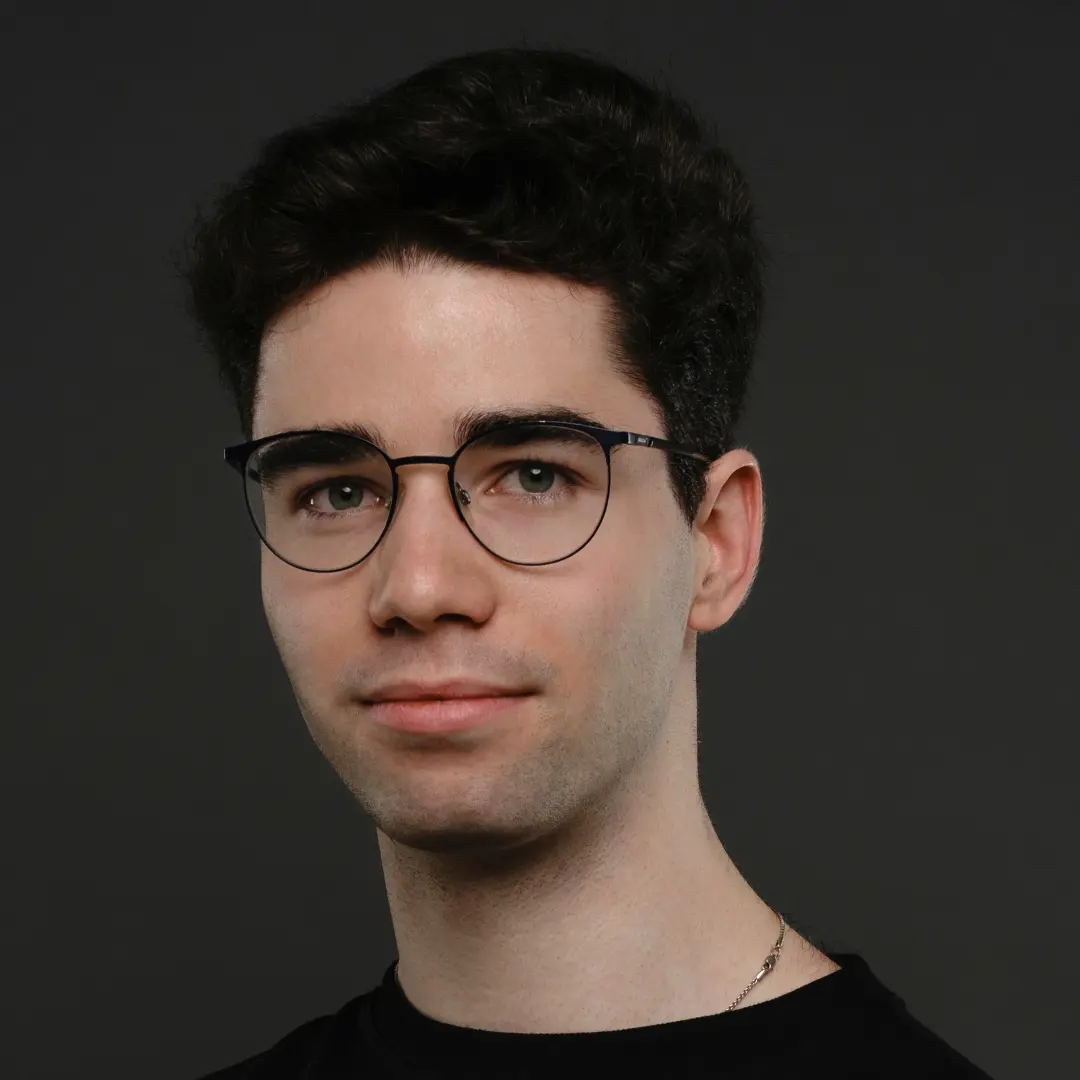
by Kyryl Sidak
Data Scientist, ML Engineer
Dec, 2023γ»7 min read
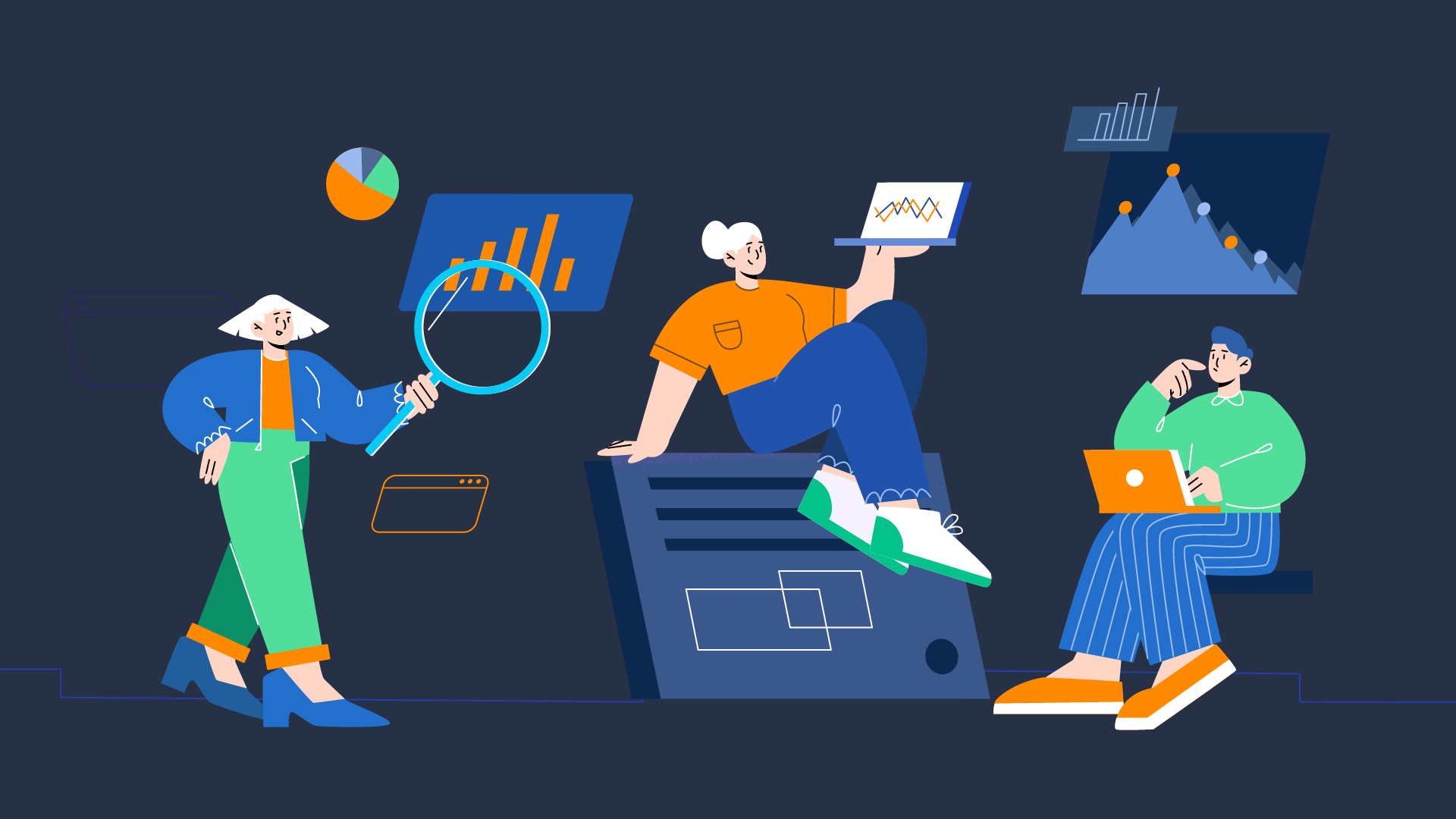
Top 3 SQL Certifications
How to Confirm Your SQL Skills
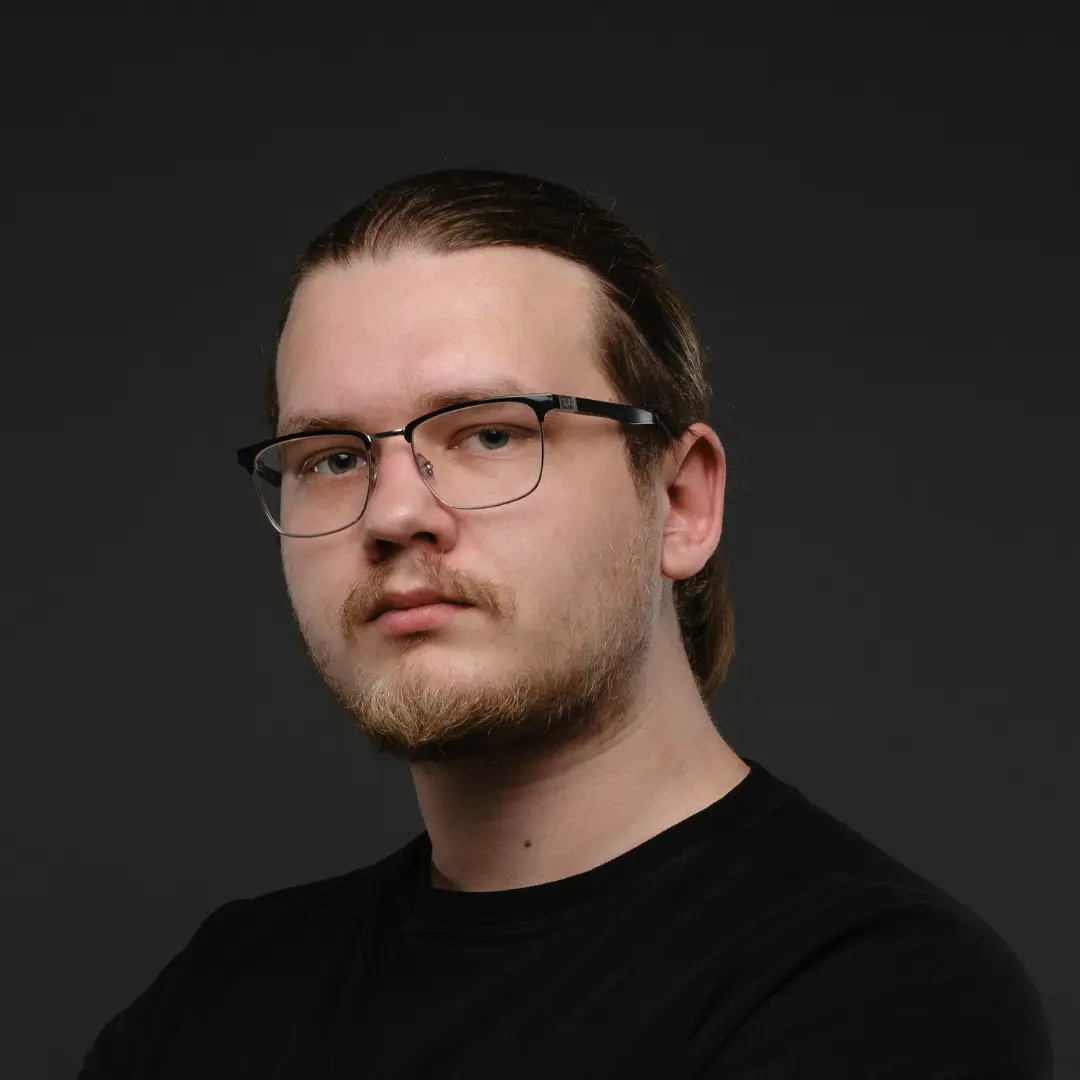
by Daniil Lypenets
Full Stack Developer
Sep, 2023γ»9 min read
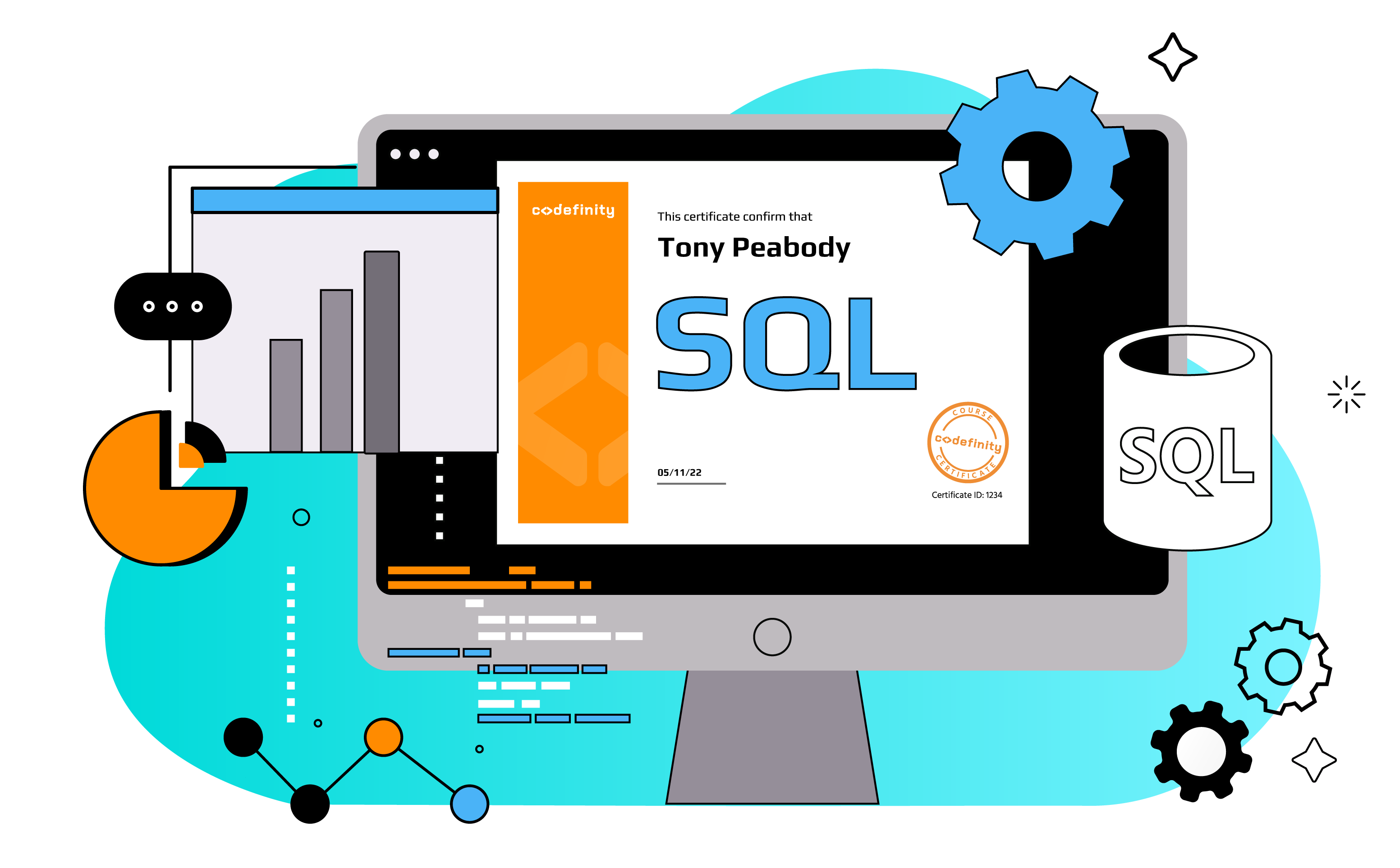
10 Essential Python Libraries Every Data Scientist Should Master
Python Libraries for Data Science
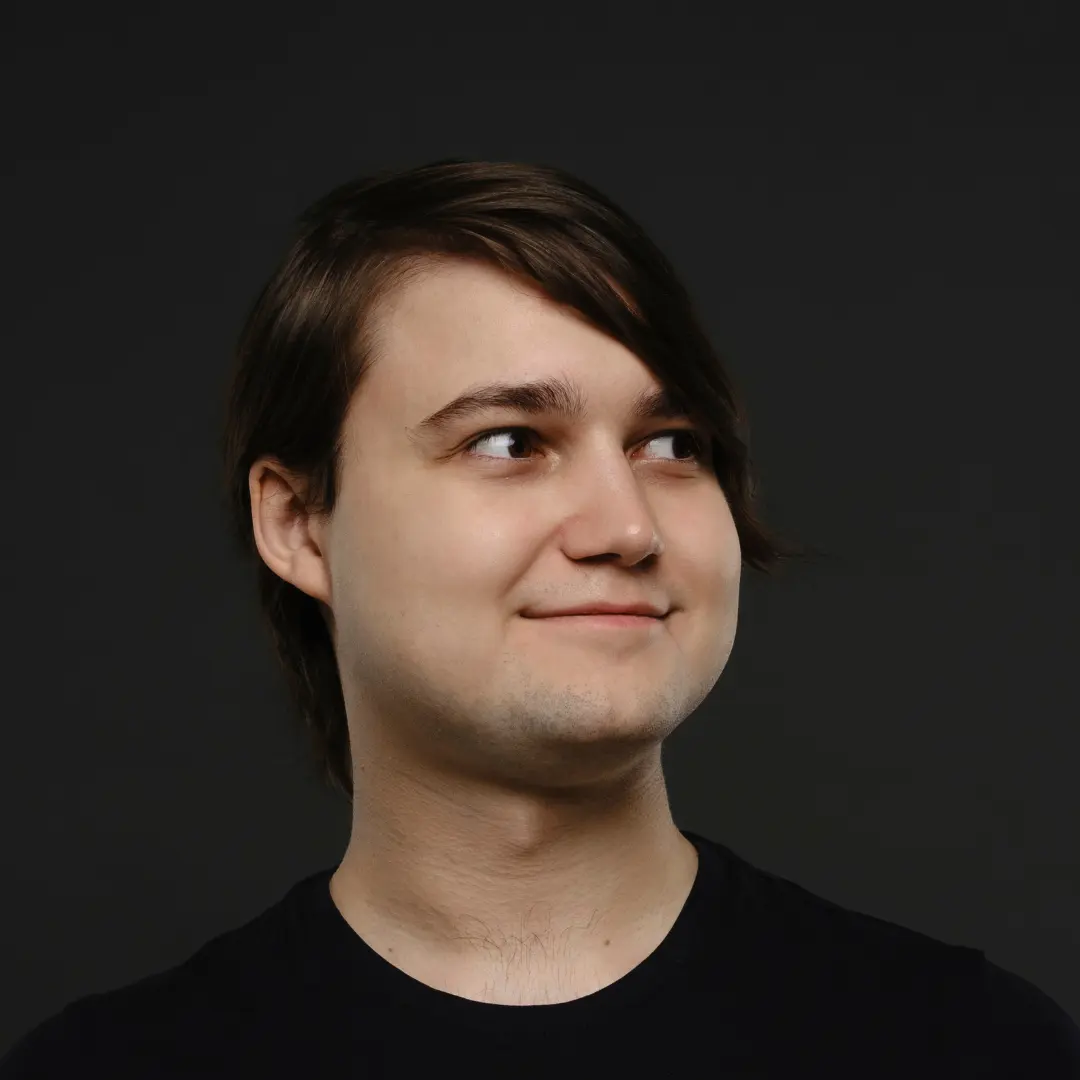
by Andrii Chornyi
Data Scientist, ML Engineer
Nov, 2023γ»7 min read
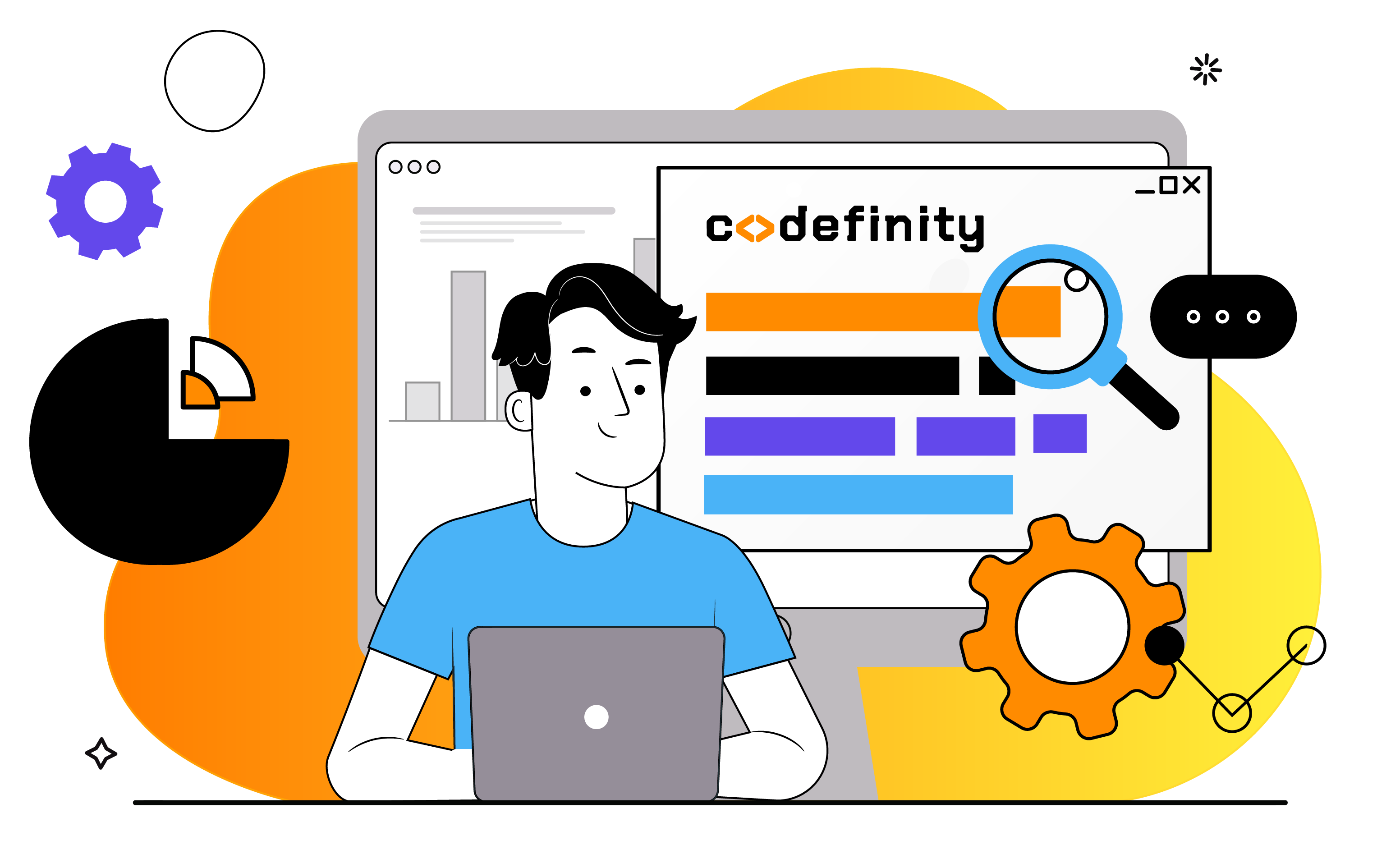
Content of this article